A Histogram Is A Graphical Representation Of
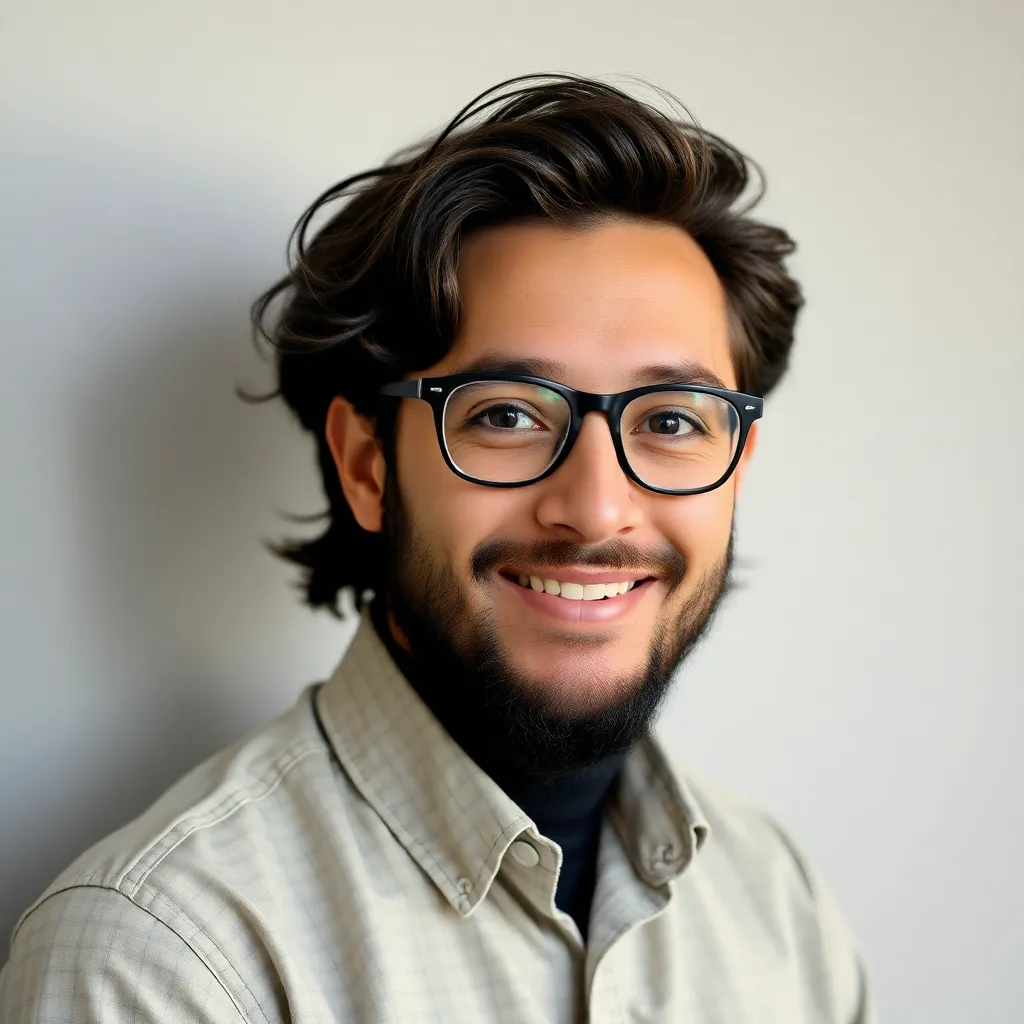
News Co
May 04, 2025 · 7 min read

Table of Contents
A Histogram: A Graphical Representation of Data Distribution
Histograms are powerful visual tools used to represent the distribution of numerical data. They provide a clear and concise way to understand the frequency of different values within a dataset, revealing patterns, trends, and outliers that might be missed in a simple data table. This comprehensive guide will explore histograms in detail, covering their construction, interpretation, and various applications across different fields.
Understanding the Basics of Histograms
At its core, a histogram is a type of bar graph that displays the frequency distribution of continuous data. Unlike bar charts that represent categorical data with distinct gaps between bars, the bars in a histogram are contiguous, representing ranges or intervals of the continuous variable. The height of each bar corresponds to the frequency or count of data points falling within that specific interval.
Key Components of a Histogram
- X-axis (Horizontal Axis): Represents the range of values of the continuous variable being measured. This axis is divided into intervals or bins.
- Y-axis (Vertical Axis): Represents the frequency or count of data points within each interval. This could also be represented as a relative frequency (percentage) or density.
- Bins (Intervals): The data is divided into a number of consecutive, non-overlapping intervals called bins. The width of each bin can be equal or unequal, depending on the data and the desired level of detail.
- Bars: Rectangular bars represent the frequency of data points within each bin. The height of the bar is proportional to the frequency.
Constructing a Histogram: A Step-by-Step Guide
Creating an effective histogram involves several crucial steps:
1. Determine the Range of Your Data
First, identify the minimum and maximum values in your dataset. This defines the overall range of your data.
2. Choose the Number of Bins
The number of bins significantly impacts the histogram's appearance and interpretation. Too few bins can obscure important details, while too many can create a jagged and uninformative graph. There's no single "correct" number of bins. Common rules of thumb include Sturges' formula (k = 1 + 3.322 * log₁₀(n), where n is the number of data points) and the square root rule (k = √n). However, experimentation and visual inspection are often necessary to find the optimal number of bins for your specific dataset.
3. Determine the Width of Each Bin
Once the number of bins is chosen, calculate the width of each bin by dividing the range of the data by the number of bins. For instance, if the range is 50 and you have 10 bins, each bin will have a width of 5.
4. Count the Frequency for Each Bin
Tally the number of data points that fall within each bin. This will give you the frequency for each interval.
5. Create the Histogram
Using the calculated frequencies, construct the histogram. Draw the x-axis representing the data range, divided into bins. Then, draw the y-axis representing the frequencies. Finally, draw rectangular bars above each bin, with the height of each bar corresponding to its frequency.
Interpreting Histograms: Unveiling Data Patterns
Once constructed, the histogram reveals valuable insights about the data distribution:
1. Central Tendency:
Observe the location of the highest bars. This indicates the center or mode of the distribution. The mode is the value that appears most frequently. You can also infer the approximate mean (average) and median (middle value) by observing the overall shape of the distribution.
2. Dispersion (Spread):
Examine the spread or variability of the data. A wide spread indicates high variability, while a narrow spread suggests low variability. The range (difference between maximum and minimum values) and standard deviation (a measure of dispersion around the mean) can be estimated visually.
3. Skewness:
Assess the symmetry of the distribution. A symmetrical distribution is roughly mirror-imaged around the center. A skewed distribution has a tail extending to one side. A right-skewed distribution has a long tail to the right, indicating a few high values, while a left-skewed distribution has a long tail to the left, suggesting a few low values.
4. Outliers:
Identify any unusual or extreme values that lie far from the majority of the data points. These outliers can significantly affect the overall shape of the histogram and might require further investigation.
5. Modality:
Determine the number of peaks or modes in the distribution. A unimodal distribution has one peak, while a bimodal distribution has two peaks, and so on. Multiple modes often suggest distinct subgroups within the data.
Applications of Histograms Across Different Fields
Histograms are versatile tools with applications across numerous fields:
1. Quality Control:
In manufacturing and industrial processes, histograms are crucial for monitoring product quality. By analyzing the distribution of a specific measurement (e.g., weight, diameter), manufacturers can identify deviations from the desired specifications and implement necessary adjustments to improve quality control.
2. Data Analysis in Research:
Histograms are extensively used in various research fields, including medicine, economics, and social sciences, to visualize and interpret data patterns. They help researchers understand the distribution of variables, identify potential outliers, and assess the reliability of their findings.
3. Financial Markets:
In finance, histograms are used to visualize the distribution of asset returns, helping investors assess risk and make informed decisions. The shape of the histogram can indicate the potential for extreme events (e.g., market crashes or booms).
4. Image Processing:
In image processing and computer vision, histograms are used to analyze the distribution of pixel intensities. This information can be used for image enhancement, contrast adjustment, and other image manipulation techniques.
5. Environmental Science:
Histograms help analyze environmental data such as pollution levels, weather patterns, and ecological distributions. They provide visual representations of data trends, enabling researchers to monitor changes and make informed predictions.
Choosing the Right Bin Width: Impact on Histogram Interpretation
The choice of bin width significantly impacts the visual representation of the data. A narrow bin width may reveal fine details but could result in a jagged histogram with high variability. Conversely, a wide bin width might smooth out the details, potentially masking important patterns. The optimal bin width often involves a trade-off between detail and clarity.
Experimentation and iterative refinement are crucial. Start with a reasonable number of bins based on a rule of thumb (like Sturges' formula), then adjust the bin width and observe the effect on the histogram's appearance. The goal is to create a histogram that provides a clear and informative representation of the data distribution, without being overly complex or simplistic.
Advanced Histogram Techniques: Density Histograms and Kernel Density Estimation
While basic histograms are straightforward to create and interpret, more advanced techniques can provide richer insights:
1. Density Histograms:
Density histograms normalize the frequencies by the bin width, presenting the data as a probability density function. This allows for direct comparison of histograms with different bin widths or datasets with different sample sizes. The area under the density histogram represents the total probability (which is 1).
2. Kernel Density Estimation (KDE):
KDE is a non-parametric method used to estimate the probability density function of a random variable. It provides a smoother representation of the data distribution compared to basic histograms, effectively reducing the impact of the arbitrary binning choices. KDE creates a continuous curve that fits the data, allowing for a more detailed visualization of the underlying distribution.
Conclusion: The Power of Visual Data Representation
Histograms are fundamental tools for visualizing and interpreting numerical data. Their ability to clearly depict the distribution, central tendency, spread, and other characteristics makes them essential for various fields, including data analysis, quality control, research, and finance. By mastering the techniques of histogram construction and interpretation, one can effectively extract meaningful insights from data and make informed decisions based on visual representations of data patterns. Remember that selecting an appropriate number of bins and understanding the limitations of histograms are crucial for effective data analysis and meaningful communication of insights. Continuous practice and careful consideration of the context are key to leveraging the full potential of histograms in data exploration and communication.
Latest Posts
Latest Posts
-
Calculating Slope From Two Points Worksheet
May 04, 2025
-
Determine The Area Of The Given Region Under The Curve
May 04, 2025
-
What Decimal Is Equivalent To 25 100
May 04, 2025
-
What Is The Y Coordinate Of Any Point On The X Axis
May 04, 2025
-
Equidistant From The Sides Of A Triangle
May 04, 2025
Related Post
Thank you for visiting our website which covers about A Histogram Is A Graphical Representation Of . We hope the information provided has been useful to you. Feel free to contact us if you have any questions or need further assistance. See you next time and don't miss to bookmark.