A Random Variable Is Said To Be Continuous If It
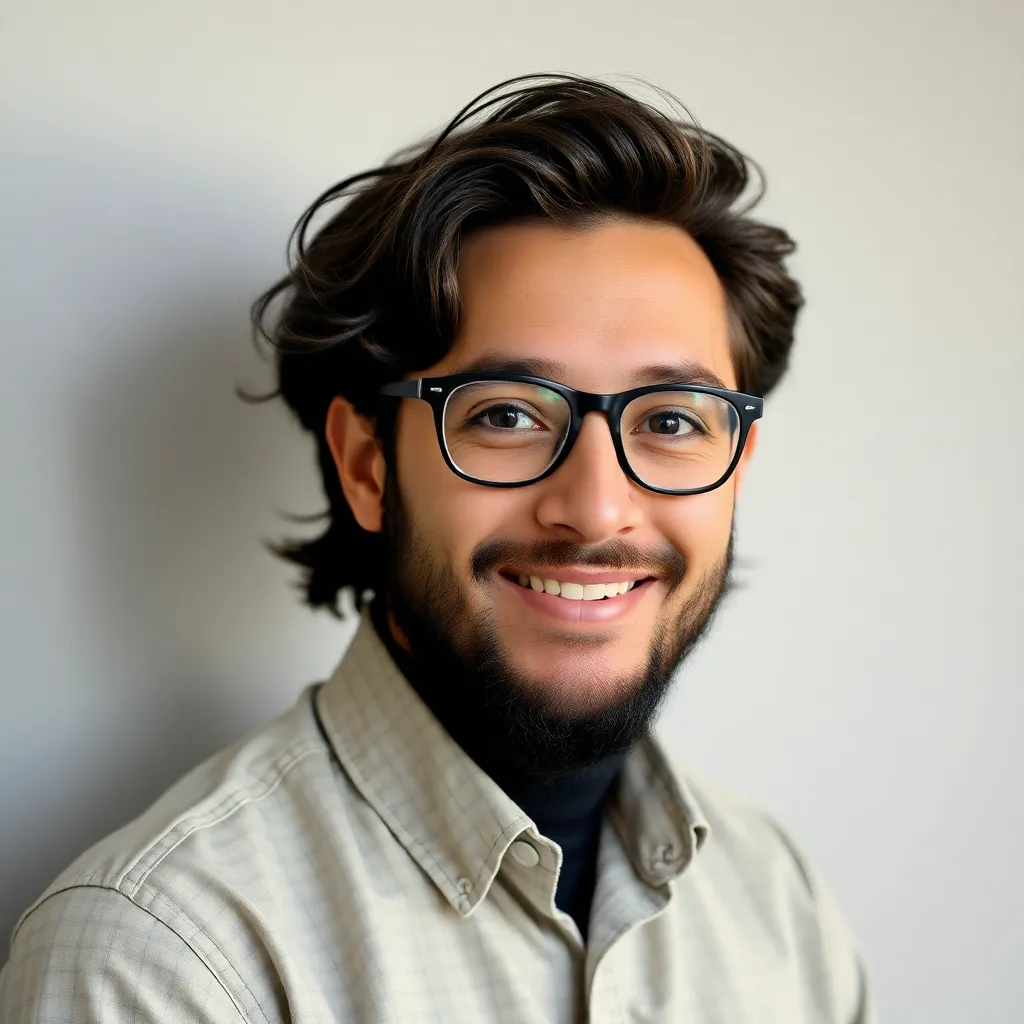
News Co
May 08, 2025 · 6 min read
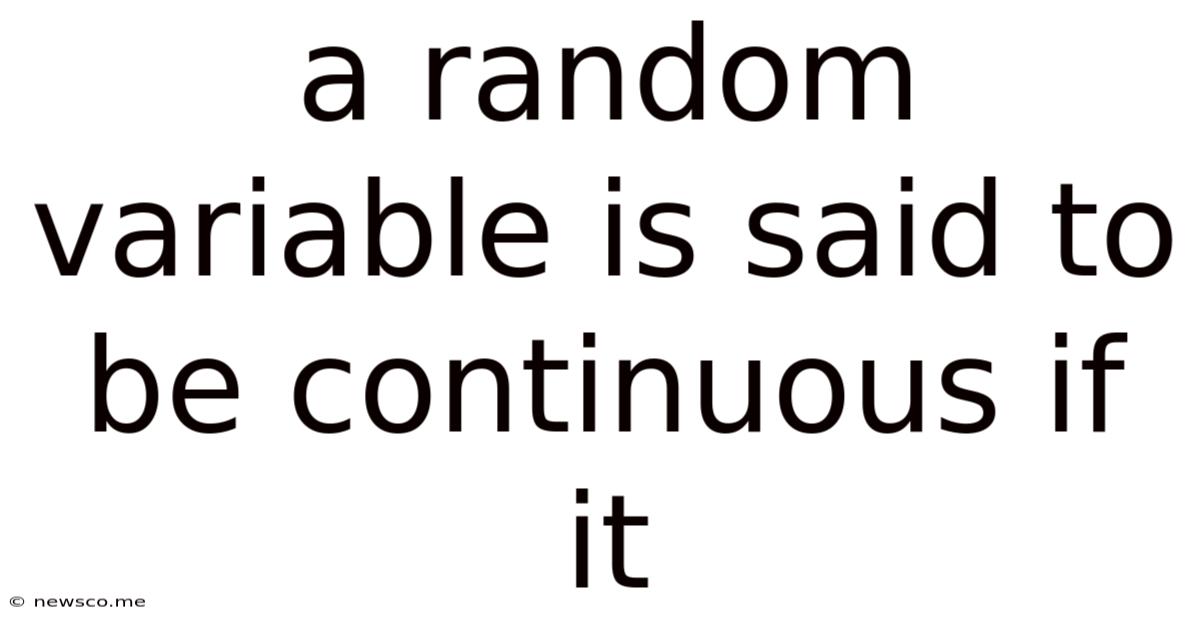
Table of Contents
A Random Variable is Said to Be Continuous If…
A random variable is a variable whose value is a numerical outcome of a random phenomenon. Understanding the difference between continuous and discrete random variables is crucial in statistics and probability. This article delves deep into the definition of a continuous random variable, exploring its properties, contrasting it with discrete variables, and providing illustrative examples. We'll also touch upon the importance of understanding continuous variables in various fields.
Defining a Continuous Random Variable
A random variable is said to be continuous if it can take on any value within a given range. Unlike discrete variables which can only take on specific, separate values (like the number of heads in three coin tosses), a continuous variable can assume an infinite number of values within an interval. This means there are no "gaps" between possible values.
Think of it this way: if you can measure a variable to an arbitrary level of precision, and still find potential values between any two measurements, it’s likely continuous. The precision is only limited by your measuring instrument, not by the inherent nature of the variable itself.
Key Characteristics of Continuous Random Variables
- Infinite Possible Values: The defining characteristic. Within a given interval, an infinite number of values are possible.
- Probability Density Function (PDF): Instead of a probability mass function (like with discrete variables), continuous variables are described by a probability density function (PDF). The PDF, denoted as f(x), doesn't give the probability of a specific value but rather the relative likelihood of the variable falling within a particular range. The probability of the variable taking on any single value is technically zero.
- Cumulative Distribution Function (CDF): The CDF, denoted as F(x), gives the probability that the random variable X is less than or equal to a given value x. This is useful for calculating probabilities within specific intervals. It's defined as the integral of the PDF.
- Integration for Probability Calculations: Probabilities are calculated by integrating the PDF over the interval of interest. This is a key difference from discrete variables where probabilities are summed.
Contrasting Continuous and Discrete Random Variables
Let's highlight the core differences between continuous and discrete random variables using a table:
Feature | Continuous Random Variable | Discrete Random Variable |
---|---|---|
Values | Can take on any value within a given range | Can only take on specific, separate values |
Number of Values | Infinite within a given range | Finite or countably infinite |
Probability of a Single Value | Zero | Non-zero |
Probability Calculation | Integration of the Probability Density Function (PDF) | Summation of the Probability Mass Function (PMF) |
Examples | Height, weight, temperature, time, voltage | Number of cars in a parking lot, number of heads in coin tosses |
Examples of Continuous Random Variables
Numerous real-world phenomena are modeled using continuous random variables. Here are a few examples across various disciplines:
1. Physical Measurements:
- Height and Weight: Human height and weight are continuous. You can measure someone's height to increasingly finer levels of precision (centimeters, millimeters, micrometers, etc.), always finding potential values between any two measurements.
- Temperature: Temperature is a classic example. The temperature can be 20°C, 20.1°C, 20.01°C, and so on. There are infinitely many possible values between any two temperatures.
- Length and Area: Measurements of length, width, area, and volume are all continuous.
- Time: Time is continuous; you can always find a smaller unit of time between any two given points in time.
2. Engineering and Physics:
- Voltage: The voltage in an electrical circuit can take on any value within a range.
- Speed and Velocity: Speed and velocity are continuous variables. A car can travel at 60 km/h, 60.1 km/h, or any value in between.
- Pressure and Density: Pressure and density are physical properties that are continuous.
3. Finance and Economics:
- Stock Prices: Although stock prices are often quoted in discrete increments (e.g., cents), the underlying price is continuous. The price can theoretically take on any value within a range.
- Interest Rates: Interest rates can fluctuate continuously over time.
- Exchange Rates: Similar to interest rates, exchange rates can take on an infinite number of values.
4. Medical Science:
- Blood Pressure: Blood pressure is a continuous variable, measured in millimeters of mercury (mmHg) or similar units.
- Body Temperature: While often measured in discrete units (e.g., degrees Celsius), body temperature is actually continuous.
The Importance of Understanding Continuous Random Variables
Understanding continuous random variables is fundamental to many fields. Here's why:
- Modeling Real-World Phenomena: Continuous variables are essential for creating realistic mathematical models of many real-world processes and systems.
- Statistical Inference: Many statistical methods, such as hypothesis testing and regression analysis, rely on the properties of continuous random variables.
- Probability Calculations: Understanding how to calculate probabilities using integration of the PDF is critical for making informed decisions based on probabilistic data.
- Data Analysis: Many data analysis techniques involve working with continuous data, requiring an understanding of continuous probability distributions.
- Simulation and Modeling: Continuous random variables are frequently used in simulations to model complex systems and processes.
Common Continuous Probability Distributions
Several common continuous probability distributions are frequently used to model continuous random variables. These include:
- Normal Distribution: Arguably the most important continuous distribution, characterized by its bell-shaped curve. It's widely used in many fields due to the Central Limit Theorem.
- Exponential Distribution: Often used to model the time between events in a Poisson process (events occurring randomly at a constant average rate).
- Uniform Distribution: A distribution where all values within a given interval are equally likely.
- Gamma Distribution: A versatile distribution used to model various phenomena, including waiting times and sums of exponential variables.
- Beta Distribution: Often used to model probabilities or proportions.
- Weibull Distribution: Used extensively in reliability engineering to model the lifetime of equipment or systems.
Each of these distributions has its own specific properties and applications, making them powerful tools for modeling and analyzing data involving continuous random variables.
Conclusion
Continuous random variables represent a crucial aspect of probability and statistics. Their ability to model a wide array of real-world phenomena makes them invaluable in various fields. By understanding their defining characteristics, contrasting them with discrete variables, and appreciating the role of the PDF and CDF, you can effectively work with and interpret data involving continuous random variables, ultimately leading to more accurate analyses and informed decision-making. The examples provided offer a glimpse into the wide-ranging applicability of this concept, underscoring its importance in both theoretical and practical contexts. Further exploration into specific continuous distributions and their applications will deepen your understanding and broaden your capabilities in this crucial area of statistical analysis.
Latest Posts
Related Post
Thank you for visiting our website which covers about A Random Variable Is Said To Be Continuous If It . We hope the information provided has been useful to you. Feel free to contact us if you have any questions or need further assistance. See you next time and don't miss to bookmark.