Among The Examples Below The Strongest Correlation Coefficient Is
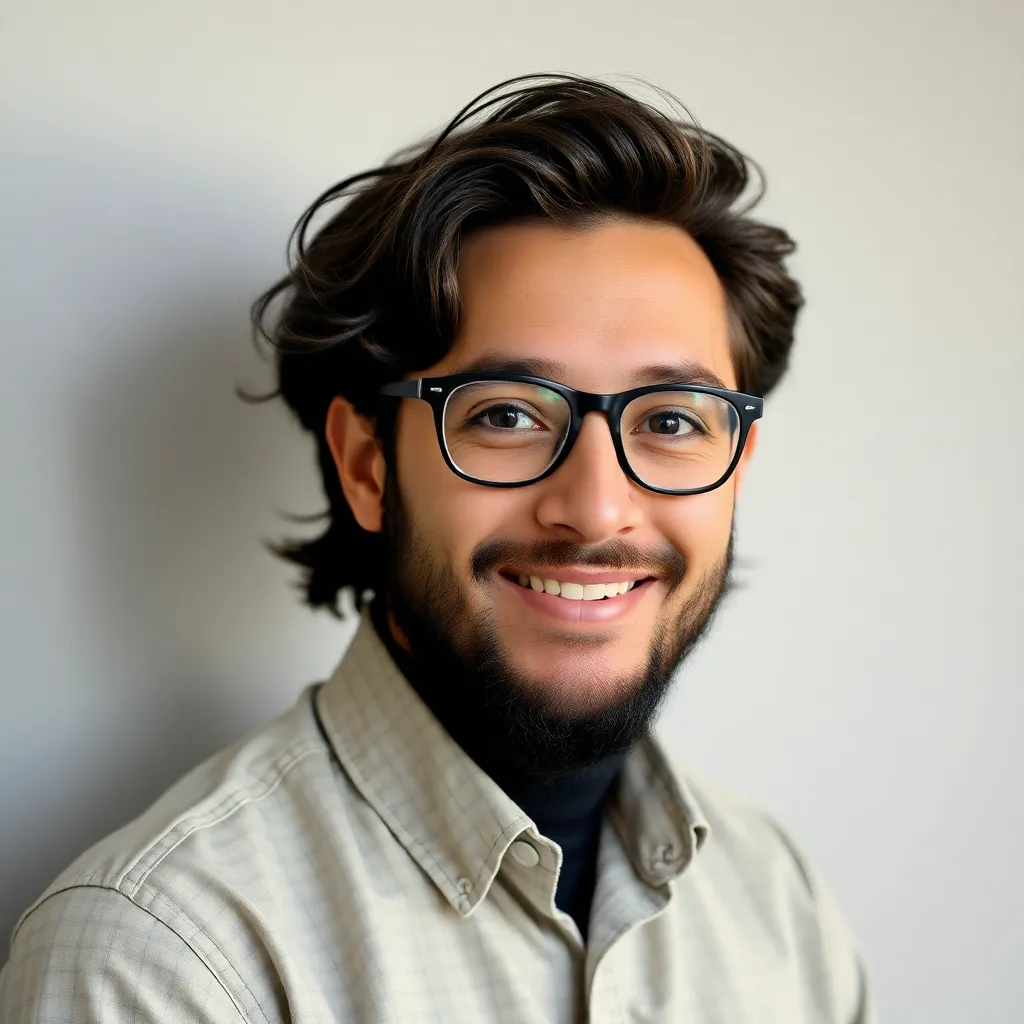
News Co
May 03, 2025 · 5 min read

Table of Contents
Among the Examples Below, the Strongest Correlation Coefficient Is: Understanding Correlation and its Strength
Correlation coefficients are fundamental statistical measures that quantify the strength and direction of a linear relationship between two variables. Understanding how to interpret these coefficients is crucial in various fields, from social sciences and economics to engineering and medicine. This article delves into the concept of correlation, different types of correlation coefficients, and how to determine which among several examples exhibits the strongest correlation. We'll explore practical applications and potential pitfalls to avoid when interpreting correlation results.
What is a Correlation Coefficient?
A correlation coefficient is a numerical value ranging from -1 to +1 that indicates the strength and direction of a linear relationship between two variables. The closer the absolute value of the coefficient is to 1, the stronger the relationship. The sign (+ or -) indicates the direction of the relationship:
- Positive Correlation (+1): As one variable increases, the other variable also increases. This represents a direct relationship.
- Negative Correlation (-1): As one variable increases, the other variable decreases. This represents an inverse relationship.
- Zero Correlation (0): There is no linear relationship between the two variables. This doesn't necessarily mean there's no relationship at all, just that it's not linear.
Several types of correlation coefficients exist, each suited for different data types:
Types of Correlation Coefficients
-
Pearson's r: This is the most common correlation coefficient, used for measuring the linear association between two continuous variables. It assumes a normal distribution of data. It's sensitive to outliers, meaning a few extreme data points can significantly influence the result.
-
Spearman's rank correlation (ρ): This non-parametric coefficient measures the monotonic relationship between two variables. It's less sensitive to outliers than Pearson's r and can be used with ordinal data (ranked data). It assesses whether the ranks of the variables increase or decrease together, regardless of the exact numerical values.
-
Kendall's tau (τ): Another non-parametric correlation coefficient that measures the ordinal association between two variables. It's less sensitive to outliers than Pearson's r and is often preferred when dealing with small datasets or datasets with many tied ranks.
-
Point-Biserial Correlation: Used when one variable is continuous and the other is dichotomous (only two categories).
-
Phi Coefficient: Used when both variables are dichotomous.
Identifying the Strongest Correlation Coefficient: Examples
Let's consider some hypothetical examples to illustrate how to determine the strongest correlation coefficient:
Example 1:
- Variables: Hours of study and exam scores.
- Correlation Coefficient (Pearson's r): 0.85
Example 2:
- Variables: Ice cream sales and crime rate.
- Correlation Coefficient (Pearson's r): 0.70
Example 3:
- Variables: Temperature and coat sales.
- Correlation Coefficient (Pearson's r): -0.92
Example 4:
- Variables: Height and shoe size.
- Correlation Coefficient (Spearman's ρ): 0.80
Analysis:
In this set of examples, the strongest correlation is represented by Example 3, with a Pearson's r of -0.92. While Example 1 shows a strong positive correlation (0.85), the absolute value of -0.92 is higher, indicating a stronger linear relationship between temperature and coat sales (an inverse relationship). Example 4, using Spearman's ρ, shows a strong correlation (0.80), but the strongest correlation is based on the highest absolute value among all examples.
Interpreting the Strength of Correlation
The strength of correlation is often categorized as follows:
- Strong: |r| ≥ 0.7
- Moderate: 0.5 ≤ |r| < 0.7
- Weak: 0.3 ≤ |r| < 0.5
- Negligible or No Correlation: |r| < 0.3
Important Note: A strong correlation does not imply causation. Just because two variables are strongly correlated doesn't mean one causes the other. There might be a third, lurking variable influencing both. For instance, in the ice cream sales and crime rate example (Example 2), both variables might be influenced by a third variable: hot weather.
Practical Applications of Correlation Analysis
Correlation analysis is widely used in various fields:
- Finance: Assessing the relationship between different financial assets.
- Marketing: Determining the effectiveness of advertising campaigns.
- Medicine: Investigating the relationship between lifestyle factors and disease risk.
- Engineering: Studying the relationship between different design parameters and product performance.
- Social Sciences: Examining the correlation between social factors and various outcomes.
Potential Pitfalls and Considerations
-
Outliers: Outliers can disproportionately affect correlation coefficients, especially Pearson's r. It's essential to identify and address outliers appropriately. Methods include removing them (with caution) or using robust correlation methods.
-
Non-linear Relationships: Correlation coefficients primarily measure linear relationships. If the relationship between variables is non-linear (e.g., curvilinear), the correlation coefficient might underestimate the strength of the relationship. Visualizing the data using scatter plots is crucial to assess this.
-
Causation vs. Correlation: As mentioned earlier, correlation does not equal causation. Careful consideration of potential confounding variables is necessary to avoid drawing incorrect causal inferences.
-
Sample Size: The reliability of a correlation coefficient depends on the sample size. Larger sample sizes generally lead to more reliable estimates.
-
Data Type: Selecting the appropriate correlation coefficient for the data type (continuous, ordinal, dichotomous) is crucial for accurate results.
Advanced Techniques and Further Exploration
For more complex analyses, consider exploring:
-
Partial Correlation: This technique assesses the correlation between two variables while controlling for the effects of a third (or more) variable.
-
Multiple Regression Analysis: This allows you to examine the relationship between a dependent variable and multiple independent variables simultaneously.
-
Canonical Correlation Analysis: This technique investigates the relationships between two sets of variables.
Conclusion
Determining the strongest correlation coefficient among several examples involves comparing the absolute values of the coefficients. The higher the absolute value (closer to 1), the stronger the linear relationship between the two variables. However, it's crucial to remember that correlation does not imply causation, and careful interpretation, considering the context and potential limitations, is essential for drawing meaningful conclusions. By understanding the different types of correlation coefficients, their strengths and limitations, and applying appropriate statistical methods, researchers can accurately assess and interpret the relationships between variables, leading to better insights and informed decision-making across various fields. Always visualize your data using scatter plots to confirm the linearity assumption and detect potential outliers before drawing conclusions. Remember to consider the type of data and choose the appropriate correlation measure. Finally, always be cautious in interpreting correlation; a strong correlation doesn't necessarily indicate a causal relationship.
Latest Posts
Latest Posts
-
All Values At Which Has A Local Maximum
May 04, 2025
-
11 2 3 As An Improper Fraction
May 04, 2025
-
A Chord That Passes Through The Center Of The Circle
May 04, 2025
-
Is An Isosceles Triangle A Right Triangle
May 04, 2025
-
How Many Kilograms Is 70 Lbs
May 04, 2025
Related Post
Thank you for visiting our website which covers about Among The Examples Below The Strongest Correlation Coefficient Is . We hope the information provided has been useful to you. Feel free to contact us if you have any questions or need further assistance. See you next time and don't miss to bookmark.