Difference Between F Test And T Test
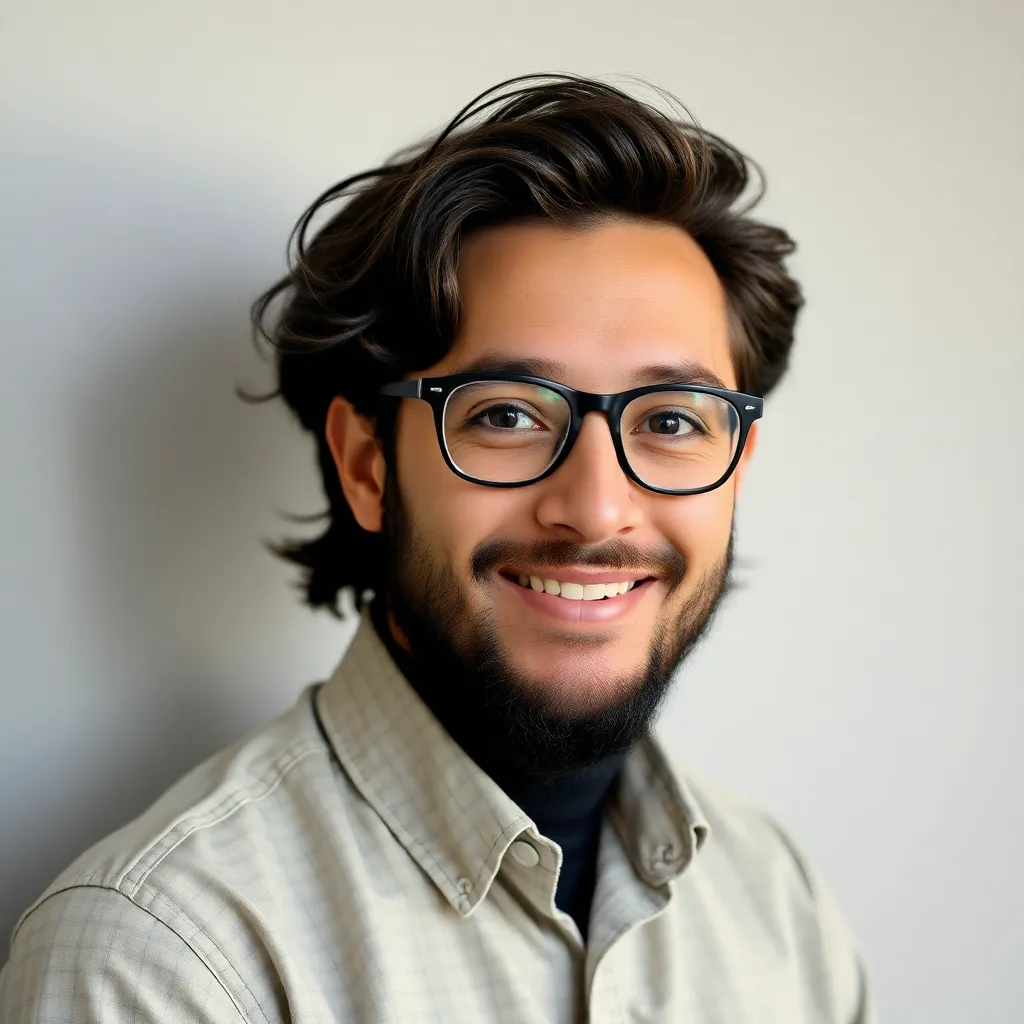
News Co
May 04, 2025 · 6 min read

Table of Contents
F-test vs. T-test: Unveiling the Differences and Applications
Choosing the right statistical test is crucial for accurate data analysis. Two frequently encountered tests, the F-test and the t-test, are both used to compare means, but they serve different purposes and are applicable under different circumstances. This comprehensive guide delves into the core distinctions between these tests, outlining their applications, assumptions, and interpretations. Understanding these differences will empower you to select the appropriate test for your specific research question and data.
Understanding the F-test
The F-test, named after statistician Ronald Fisher, is primarily used to compare the variances of two or more groups. It's a powerful tool for analyzing variance and determining if significant differences exist between the spread of data in different populations or samples. The core principle of the F-test lies in the ratio of variances: a larger F-statistic indicates a greater difference in variances.
Key Applications of the F-test:
-
ANOVA (Analysis of Variance): This is arguably the most common application of the F-test. ANOVA uses the F-test to determine if there's a statistically significant difference between the means of three or more groups. For example, you might use ANOVA to compare the average test scores of students taught using three different teaching methods.
-
Testing for Equality of Variances: Before conducting certain other tests (like the t-test), you might first use an F-test to check if the variances of two groups are equal. This assumption of equal variances is crucial for some statistical tests.
-
Regression Analysis: In regression models, the F-test assesses the overall significance of the model. It determines if at least one of the predictor variables is significantly related to the outcome variable.
Assumptions of the F-test:
The accuracy and reliability of the F-test depend on certain assumptions about the data:
-
Independence: Observations within and between groups should be independent. This means that the value of one observation doesn't influence the value of another.
-
Normality: The data within each group should be approximately normally distributed. While the F-test is relatively robust to violations of normality, especially with larger sample sizes, significant departures from normality can affect the results.
-
Homogeneity of Variances (for ANOVA): In ANOVA, the variances of the different groups being compared should be approximately equal. Violations of this assumption can lead to inaccurate results.
Understanding the T-test
The t-test, developed by William Sealy Gosset, is primarily used to compare the means of two groups. Unlike the F-test, which focuses on variances, the t-test focuses directly on the difference between the means. The t-statistic represents the difference between the group means relative to the variability within the groups.
Types of T-tests:
There are several types of t-tests, each designed for different situations:
-
One-sample t-test: This test compares the mean of a single group to a known or hypothesized value. For example, you might use this test to see if the average height of a sample of adults differs significantly from the known average height of the adult population.
-
Independent samples t-test (or two-sample t-test): This test compares the means of two independent groups. For example, you might use this test to compare the average test scores of students who received a new teaching method versus those who received the traditional method. This test has two variations:
- Assuming equal variances: Used when the variances of the two groups are approximately equal.
- Not assuming equal variances (Welch's t-test): Used when the variances of the two groups are significantly different.
-
Paired samples t-test: This test compares the means of two related groups. For example, you might use this test to compare the blood pressure of the same individuals before and after taking a medication. This test involves measuring the same subjects under two different conditions.
Assumptions of the T-test:
Similar to the F-test, the t-test relies on certain assumptions for reliable results:
-
Independence: Observations within each group should be independent.
-
Normality: The data within each group should be approximately normally distributed. Again, the t-test is relatively robust to violations of normality, particularly with larger sample sizes.
-
Homogeneity of Variances (for independent samples t-test): For the independent samples t-test assuming equal variances, the variances of the two groups being compared should be approximately equal. Welch's t-test doesn't require this assumption.
Key Differences Between F-test and T-test:
Feature | F-test | T-test |
---|---|---|
Purpose | Compares variances of two or more groups | Compares means of two groups |
Number of Groups | Two or more | Two |
Focus | Variance | Mean difference |
Output Statistic | F-statistic | T-statistic |
Common Use | ANOVA, testing for equality of variances | Comparing means of two groups (independent or paired) |
Assumptions | Independence, normality, homogeneity of variances (for ANOVA) | Independence, normality, homogeneity of variances (for independent samples, assuming equal variances) |
Choosing Between F-test and T-test: A Practical Guide
The choice between an F-test and a t-test depends entirely on your research question and the nature of your data.
-
If you want to compare the means of two groups: Use a t-test. Choose between an independent samples t-test (for unrelated groups) or a paired samples t-test (for related groups). Consider Welch's t-test if the variances are unequal.
-
If you want to compare the means of three or more groups: Use ANOVA, which employs the F-test.
-
If you want to test for the equality of variances between two groups: Use an F-test. This is often a preliminary step before conducting a t-test (if the t-test assumes equal variances).
-
If you're conducting regression analysis: The F-test assesses the overall significance of the model.
Interpreting Results: P-values and Significance
Both the F-test and the t-test produce a p-value. The p-value represents the probability of observing the obtained results (or more extreme results) if there were no real difference between the groups (or no relationship between variables in the case of regression). A small p-value (typically below a significance level of 0.05) suggests that the observed difference is statistically significant, meaning it's unlikely to have occurred by chance.
Advanced Considerations and Limitations
While powerful, both the F-test and t-test have limitations:
-
Sensitivity to outliers: Both tests can be sensitive to extreme values (outliers) in the data. Outliers can disproportionately influence the results, leading to inaccurate conclusions.
-
Violation of assumptions: While somewhat robust to violations of normality, significant deviations from the assumptions (especially independence and homogeneity of variances) can compromise the validity of the results. Transforming the data or using alternative non-parametric tests might be necessary in such cases.
-
Focus on means: Both tests primarily focus on comparing means. They may not capture all aspects of the data distribution or relationships between variables.
Conclusion: Selecting the Right Tool for the Job
The F-test and t-test are fundamental statistical tools used for comparing means and variances. Understanding their distinct applications, assumptions, and interpretations is vital for conducting robust and meaningful data analysis. By carefully considering the nature of your research question and the characteristics of your data, you can confidently select the appropriate test and draw accurate conclusions. Always remember to consider the limitations of these tests and explore alternative approaches if necessary. Accurate statistical analysis is critical for making sound decisions based on data, and selecting the right test is the first step in achieving this goal.
Latest Posts
Latest Posts
-
Which Of The Following Is A Singleton Set
May 04, 2025
-
10 Is 50 Of What Number
May 04, 2025
-
Is 1 2 A Rational Or Irrational Number
May 04, 2025
-
What Are The Properties Of Translation
May 04, 2025
-
Natural Numbers Are Closed Under Division
May 04, 2025
Related Post
Thank you for visiting our website which covers about Difference Between F Test And T Test . We hope the information provided has been useful to you. Feel free to contact us if you have any questions or need further assistance. See you next time and don't miss to bookmark.