Difference Between T Test And F Test
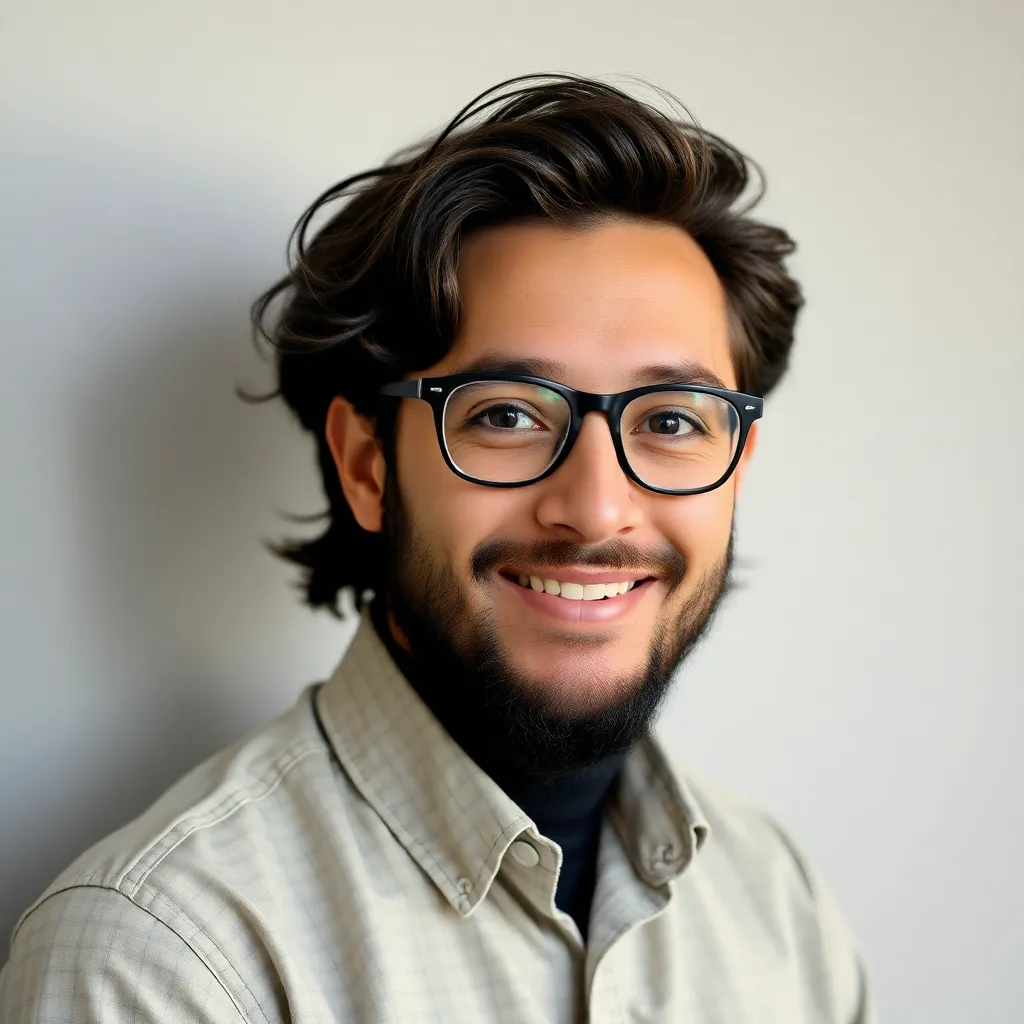
News Co
May 08, 2025 · 7 min read
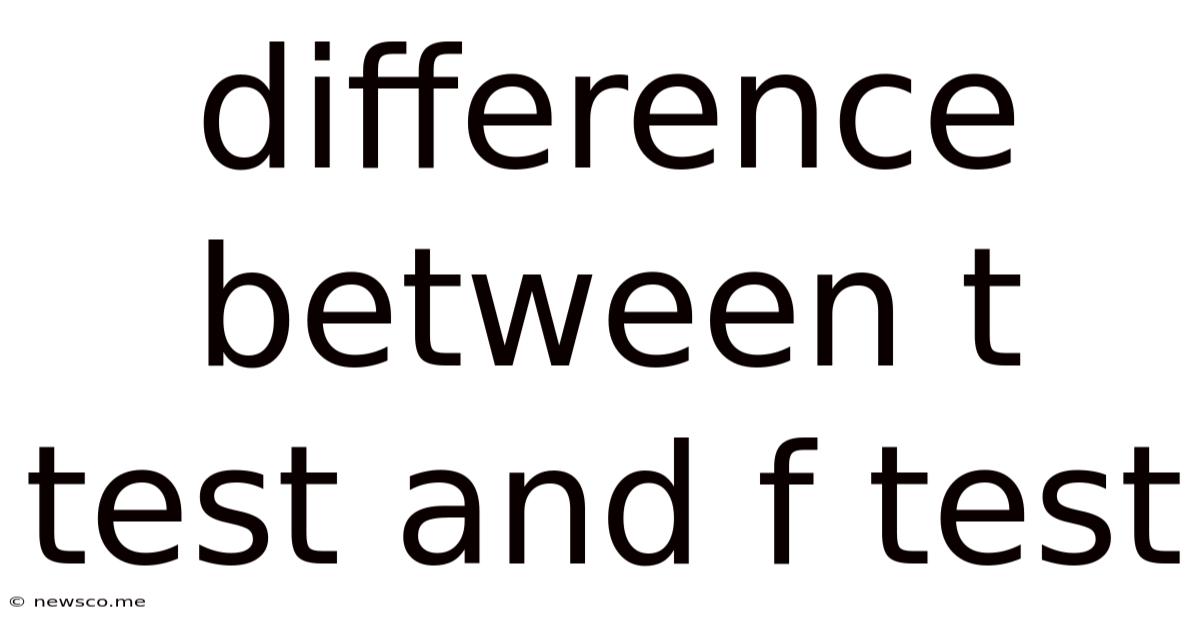
Table of Contents
Unveiling the Differences: T-Test vs. F-Test in Statistical Analysis
Both the t-test and the F-test are fundamental tools in statistical inference, used to analyze data and draw conclusions about populations based on sample data. While they both assess the significance of differences between groups, they do so under different circumstances and with different assumptions. Understanding their distinctions is crucial for selecting the appropriate test for your research question and ensuring accurate interpretation of results. This comprehensive guide will delve into the core differences between t-tests and F-tests, exploring their applications, assumptions, and interpretations.
Understanding the T-Test: A Deep Dive
The t-test is a parametric test used to compare the means of two groups. It's particularly useful when examining the difference between a sample mean and a population mean (one-sample t-test) or comparing the means of two independent or paired samples (independent samples t-test and paired samples t-test, respectively). The core of the t-test lies in the calculation of the t-statistic, which assesses the ratio of the difference between the sample means to the standard error of the difference.
Types of T-Tests:
-
One-Sample T-Test: This test determines whether a sample mean differs significantly from a known population mean. For instance, you might use this to compare the average height of a sample of students to the known average height of all students in a specific region.
-
Independent Samples T-Test: This test compares the means of two independent groups. An example would be comparing the average test scores of students who received a new teaching method versus those who received the traditional method. A crucial assumption here is that the data in each group is independently sampled and normally distributed.
-
Paired Samples T-Test: This test is used when the same subjects are measured twice, such as before and after an intervention. This approach controls for individual differences, providing a more precise comparison. For instance, you could use this to assess the effectiveness of a weight-loss program by comparing participants' weights before and after the program.
Assumptions of the T-Test:
The validity of the t-test hinges on several assumptions:
-
Normality: The data within each group should be approximately normally distributed. While the t-test is relatively robust to violations of normality, especially with larger sample sizes, significant departures can affect the accuracy of the results.
-
Independence: Observations within each group must be independent of each other. This means that one data point should not influence another.
-
Homogeneity of Variance (for Independent Samples T-Test): For independent samples t-tests, the variances of the two groups should be approximately equal. Violations of this assumption can be addressed using alternative tests like Welch's t-test, which does not assume equal variances.
Understanding the F-Test: A Powerful Tool for Variance Analysis
The F-test, named after Sir Ronald Fisher, is a statistical test used to assess the significance of differences between the means of three or more groups or to compare the variances of two or more groups. It's the cornerstone of Analysis of Variance (ANOVA), a widely used statistical technique. The F-statistic is calculated as the ratio of two variances – typically the variance between groups and the variance within groups. A larger F-statistic suggests a greater difference between group means relative to the variability within groups.
Types of F-Tests:
-
ANOVA (Analysis of Variance): ANOVA uses the F-test to compare the means of three or more independent groups. For instance, you could use ANOVA to compare the average crop yields of different fertilizers. One-way ANOVA compares means across one independent variable, while two-way ANOVA examines the effects of two or more independent variables.
-
Two-Way ANOVA: This extends one-way ANOVA by incorporating two or more independent variables and examining their main effects and interactions. It's invaluable for studying complex relationships between variables.
-
MANOVA (Multivariate Analysis of Variance): This is an extension of ANOVA that allows the comparison of means across multiple dependent variables simultaneously.
-
Testing for Equality of Variances: The F-test can also be used to compare the variances of two independent groups. This is often a preliminary step before conducting other tests, such as the independent samples t-test.
Assumptions of the F-Test:
The F-test relies on several assumptions:
-
Normality: Similar to the t-test, the data within each group should be approximately normally distributed. However, ANOVA is relatively robust to violations of normality, particularly with larger sample sizes.
-
Independence: Observations within each group should be independent.
-
Homogeneity of Variance: The variances of the groups being compared should be approximately equal. Violations of this assumption can impact the accuracy of the F-test. Tests like Levene's test are used to check for homogeneity of variance.
Key Differences Between T-Test and F-Test:
Feature | T-Test | F-Test |
---|---|---|
Purpose | Compares means of two groups | Compares means of three or more groups, or compares variances of two groups |
Number of Groups | Two | Three or more (for comparing means) |
Test Statistic | t-statistic | F-statistic |
Underlying Distribution | t-distribution | F-distribution |
Typical Applications | Comparing two treatment groups, before-after comparisons | ANOVA, comparing variances, factorial designs |
Assumptions | Normality, independence, homogeneity of variance (for independent samples) | Normality, independence, homogeneity of variance |
Choosing the Right Test: A Practical Guide
Selecting between a t-test and an F-test depends entirely on your research question and the nature of your data:
-
Two Groups, Comparing Means: Use a t-test (one-sample, independent samples, or paired samples, depending on your data structure).
-
Three or More Groups, Comparing Means: Use ANOVA (F-test).
-
Comparing Variances of Two Groups: Use an F-test specifically designed for comparing variances.
Interpreting Results: Significance and Effect Size
Both t-tests and F-tests yield p-values, which indicate the probability of observing the obtained results (or more extreme results) if there were no real difference between the groups. A small p-value (typically less than 0.05) suggests that the differences are statistically significant, meaning that it's unlikely the observed differences are due to chance alone.
However, statistical significance does not necessarily imply practical significance. Effect size measures, such as Cohen's d for t-tests and eta-squared (η²) for ANOVA, provide a quantifiable measure of the magnitude of the effect. These measures help assess the practical importance of the findings, going beyond simply declaring statistical significance.
Beyond the Basics: Advanced Considerations
The world of statistical testing extends far beyond the basic t-test and F-test. Non-parametric alternatives exist for situations where the assumptions of normality are severely violated. These include tests like the Mann-Whitney U test (analogous to the independent samples t-test) and the Kruskal-Wallis test (analogous to ANOVA). Furthermore, the choice of statistical test can be influenced by the type of data you have (e.g., continuous, categorical), the research design, and the specific questions you are trying to answer.
Conclusion: Mastering T-Tests and F-Tests for Powerful Data Analysis
The t-test and F-test are powerful tools in a statistician's arsenal. Understanding their differences, assumptions, and appropriate applications is crucial for conducting rigorous and meaningful statistical analyses. By carefully considering the nature of your data and your research question, you can select the appropriate test and interpret the results accurately, leading to sound conclusions and valuable insights from your research. Remember that mastering these tests is an iterative process; practice and a solid understanding of statistical principles are key to effective application. Consult statistical resources and seek guidance when needed to enhance your analytical skills and draw reliable inferences from your data.
Latest Posts
Latest Posts
-
What Is The Greatest Common Factor Of 96
May 08, 2025
-
2 Lines Cut By A Transversal In Real Life
May 08, 2025
-
A Number Raised To The Ninth Power
May 08, 2025
-
The Distance Around A Polygon Is Called The
May 08, 2025
-
How Much Is 270 Pounds In Us Dollars
May 08, 2025
Related Post
Thank you for visiting our website which covers about Difference Between T Test And F Test . We hope the information provided has been useful to you. Feel free to contact us if you have any questions or need further assistance. See you next time and don't miss to bookmark.