How To Find Point Estimate With Lower And Upper Bound
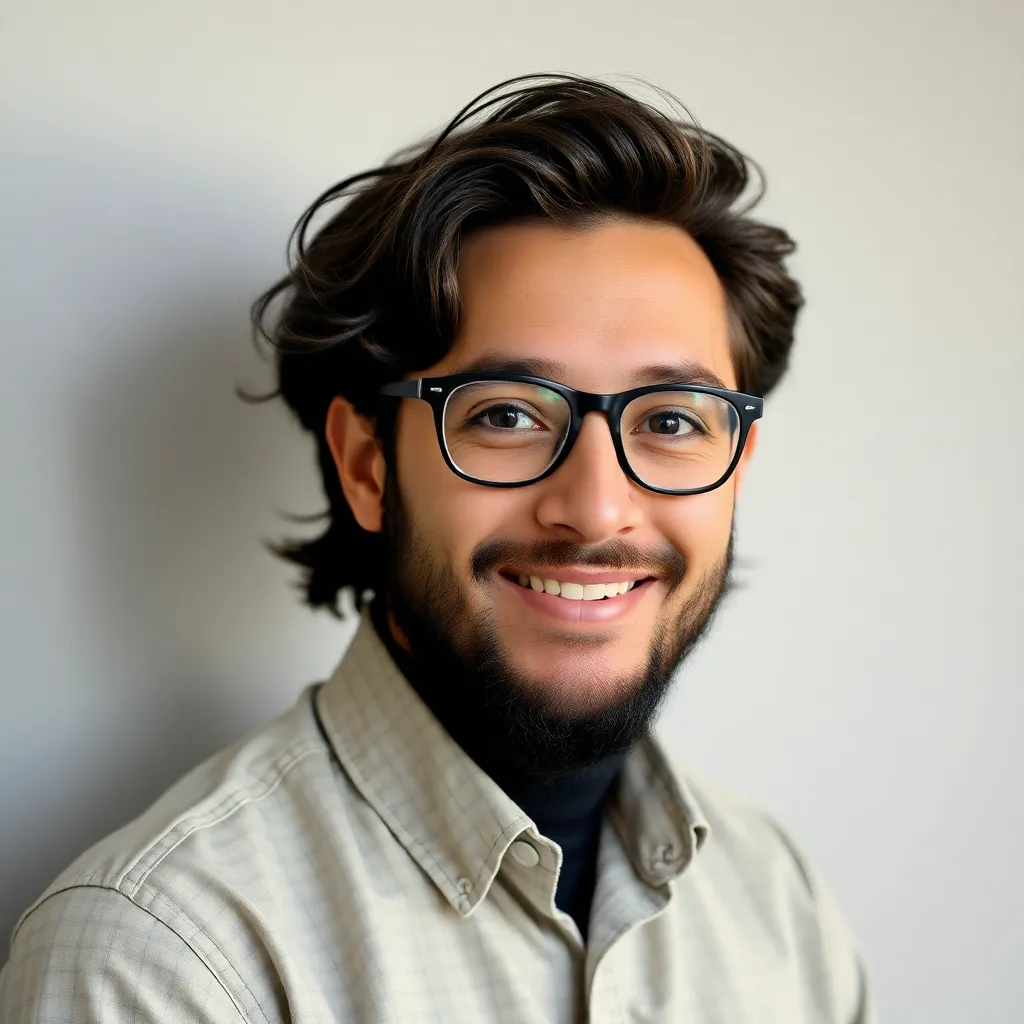
News Co
May 08, 2025 · 6 min read
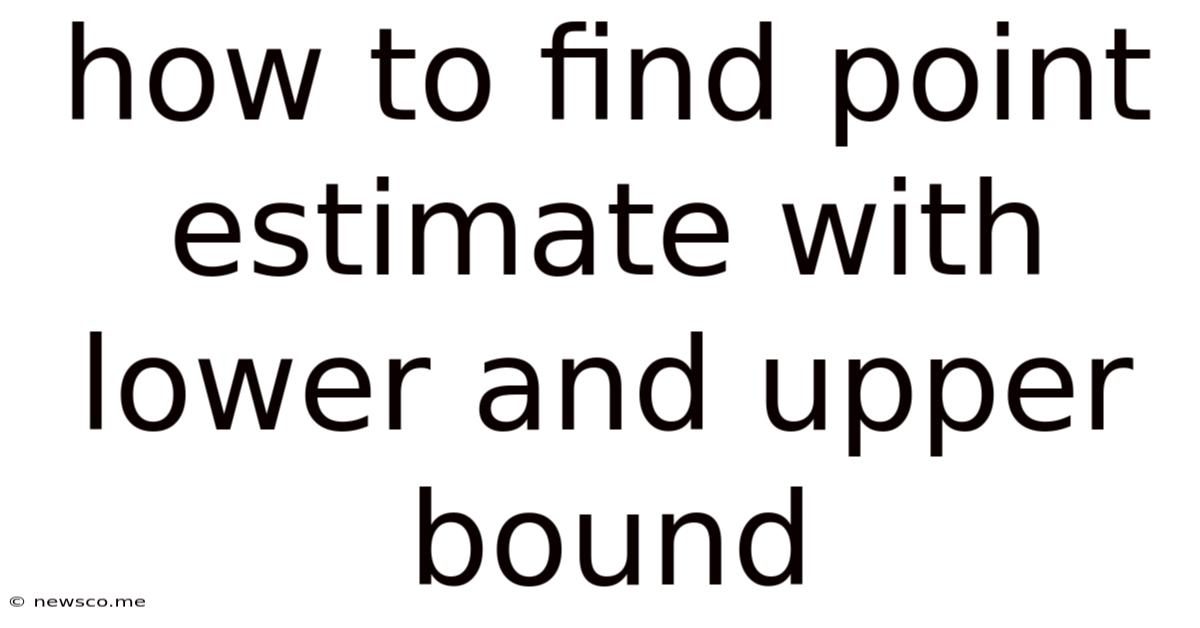
Table of Contents
How to Find Point Estimates with Lower and Upper Bounds: A Comprehensive Guide
Finding point estimates with their associated lower and upper bounds is crucial in statistical inference. It allows us to not only provide a single best guess for a population parameter (the point estimate) but also quantify the uncertainty surrounding that guess. This uncertainty is represented by the confidence interval, defined by the lower and upper bounds. This guide will delve into the methods and concepts necessary to master this essential statistical skill.
Understanding Point Estimates and Confidence Intervals
Before diving into the methods, let's clarify the core concepts:
Point Estimate: A Single Best Guess
A point estimate is a single value used to estimate an unknown population parameter. For example, we might use the sample mean (average) to estimate the population mean. While convenient, a point estimate alone doesn't convey the uncertainty inherent in using a sample to represent a population.
Confidence Interval: Quantifying Uncertainty
A confidence interval provides a range of values within which the true population parameter is likely to fall. This range is constructed around the point estimate and is expressed as a percentage (e.g., a 95% confidence interval). A higher percentage indicates a greater degree of confidence, but also a wider interval. The lower and upper bounds define the limits of this interval.
Key Terms to Remember:
- Population Parameter: The true value of the characteristic being measured in the entire population (e.g., the true average height of all adults). This is usually unknown.
- Sample Statistic: A value calculated from a sample of data (e.g., the average height of a sample of 100 adults).
- Confidence Level: The probability that the confidence interval contains the true population parameter (e.g., 95%, 99%).
- Margin of Error: The amount added and subtracted from the point estimate to obtain the lower and upper bounds of the confidence interval. A larger margin of error indicates greater uncertainty.
- Standard Error: A measure of the variability of the sample statistic. It's crucial in calculating the margin of error. A smaller standard error suggests a more precise estimate.
Methods for Finding Point Estimates and Confidence Intervals
The specific method for calculating point estimates and confidence intervals depends on the type of data you have and the parameter you're estimating. Here are some common scenarios:
1. Estimating Population Mean (μ) with Known Population Standard Deviation (σ)
When the population standard deviation is known (a rare situation in practice), we use the z-distribution to construct the confidence interval.
Point Estimate: The sample mean (x̄)
Confidence Interval: x̄ ± z * (σ / √n)
Where:
- x̄ = sample mean
- z = z-score corresponding to the desired confidence level (e.g., 1.96 for 95% confidence)
- σ = population standard deviation
- n = sample size
Example: Suppose we have a sample of 100 light bulbs with a mean lifespan of 1000 hours and a known population standard deviation of 100 hours. To calculate a 95% confidence interval:
- Point Estimate: x̄ = 1000 hours
- z-score: z = 1.96 (for 95% confidence)
- Standard Error: σ / √n = 100 / √100 = 10 hours
- Margin of Error: 1.96 * 10 = 19.6 hours
- Confidence Interval: 1000 ± 19.6 hours, or (980.4, 1019.6) hours
2. Estimating Population Mean (μ) with Unknown Population Standard Deviation (σ)
This is the more common scenario. Since σ is unknown, we estimate it using the sample standard deviation (s) and use the t-distribution instead of the z-distribution.
Point Estimate: The sample mean (x̄)
Confidence Interval: x̄ ± t * (s / √n)
Where:
- x̄ = sample mean
- t = t-score corresponding to the desired confidence level and degrees of freedom (n-1)
- s = sample standard deviation
- n = sample size
Example: Let's say we have a sample of 25 students with an average test score of 75 and a sample standard deviation of 10. To calculate a 99% confidence interval:
- Point Estimate: x̄ = 75
- t-score: With 24 degrees of freedom (25-1) and a 99% confidence level, the t-score is approximately 2.80. You'll need a t-table or statistical software to find this value.
- Standard Error: s / √n = 10 / √25 = 2
- Margin of Error: 2.80 * 2 = 5.6
- Confidence Interval: 75 ± 5.6, or (69.4, 80.6)
3. Estimating Population Proportion (p)
When estimating a population proportion (e.g., the percentage of voters who support a particular candidate), we use the following:
Point Estimate: The sample proportion (p̂) = x/n, where x is the number of successes and n is the sample size.
Confidence Interval: p̂ ± z * √[(p̂(1-p̂))/n]
Where:
- p̂ = sample proportion
- z = z-score corresponding to the desired confidence level
- n = sample size
Example: Suppose in a survey of 200 people, 120 said they prefer Brand A. To calculate a 90% confidence interval for the population proportion:
- Point Estimate: p̂ = 120/200 = 0.6
- z-score: z = 1.645 (for 90% confidence)
- Standard Error: √[(0.6 * 0.4) / 200] ≈ 0.0346
- Margin of Error: 1.645 * 0.0346 ≈ 0.0569
- Confidence Interval: 0.6 ± 0.0569, or (0.5431, 0.6569)
Factors Affecting Confidence Interval Width
The width of the confidence interval reflects the uncertainty in our estimate. Several factors influence this width:
- Confidence Level: A higher confidence level (e.g., 99% vs. 95%) leads to a wider interval because we're aiming for a higher probability of capturing the true parameter.
- Sample Size (n): A larger sample size results in a narrower interval because larger samples provide more precise estimates.
- Standard Deviation (σ or s): A larger standard deviation (more variability in the data) leads to a wider interval, reflecting greater uncertainty.
Choosing the Right Method and Interpreting Results
Selecting the appropriate method for finding point estimates and confidence intervals hinges on understanding your data and research question. Always consider:
- Type of data: Are you working with continuous data (e.g., height, weight) or categorical data (e.g., gender, preference)?
- Population parameter of interest: Are you estimating the mean, proportion, variance, or another parameter?
- Knowledge of the population standard deviation: Is the population standard deviation known or unknown?
Interpreting the results involves understanding that the confidence interval provides a range of plausible values for the population parameter, not a probability statement about the parameter itself. For example, a 95% confidence interval doesn't mean there's a 95% chance the true parameter lies within the interval; rather, it means that if we were to repeat this sampling process many times, 95% of the resulting confidence intervals would contain the true parameter.
Advanced Topics and Considerations
This guide provides a foundation for understanding point estimates and confidence intervals. More advanced topics include:
- Hypothesis testing: Using confidence intervals to test hypotheses about population parameters.
- Sample size determination: Calculating the required sample size to achieve a desired confidence interval width.
- One-sided vs. two-sided confidence intervals: Focusing on a single tail of the distribution rather than both.
- Dealing with non-normal data: Utilizing non-parametric methods when the data doesn't follow a normal distribution.
- Using statistical software: Leveraging software like R, Python (with libraries like SciPy and Statsmodels), or SPSS to perform these calculations efficiently.
Mastering the techniques for calculating point estimates and confidence intervals is essential for drawing valid conclusions from statistical data. By understanding the underlying principles and choosing the appropriate method, you can effectively communicate the uncertainty inherent in statistical inferences and make informed decisions based on your findings. Remember that accurate and precise estimates are critical for data-driven decision making, and this guide helps you achieve that goal. Continued practice and exploration of these methods will enhance your skills and deepen your understanding of statistical analysis.
Latest Posts
Latest Posts
-
What Is The Greatest Common Factor Of 96
May 08, 2025
-
2 Lines Cut By A Transversal In Real Life
May 08, 2025
-
A Number Raised To The Ninth Power
May 08, 2025
-
The Distance Around A Polygon Is Called The
May 08, 2025
-
How Much Is 270 Pounds In Us Dollars
May 08, 2025
Related Post
Thank you for visiting our website which covers about How To Find Point Estimate With Lower And Upper Bound . We hope the information provided has been useful to you. Feel free to contact us if you have any questions or need further assistance. See you next time and don't miss to bookmark.