In Inferential Statistics We Calculate Statistics Of Sample Data To
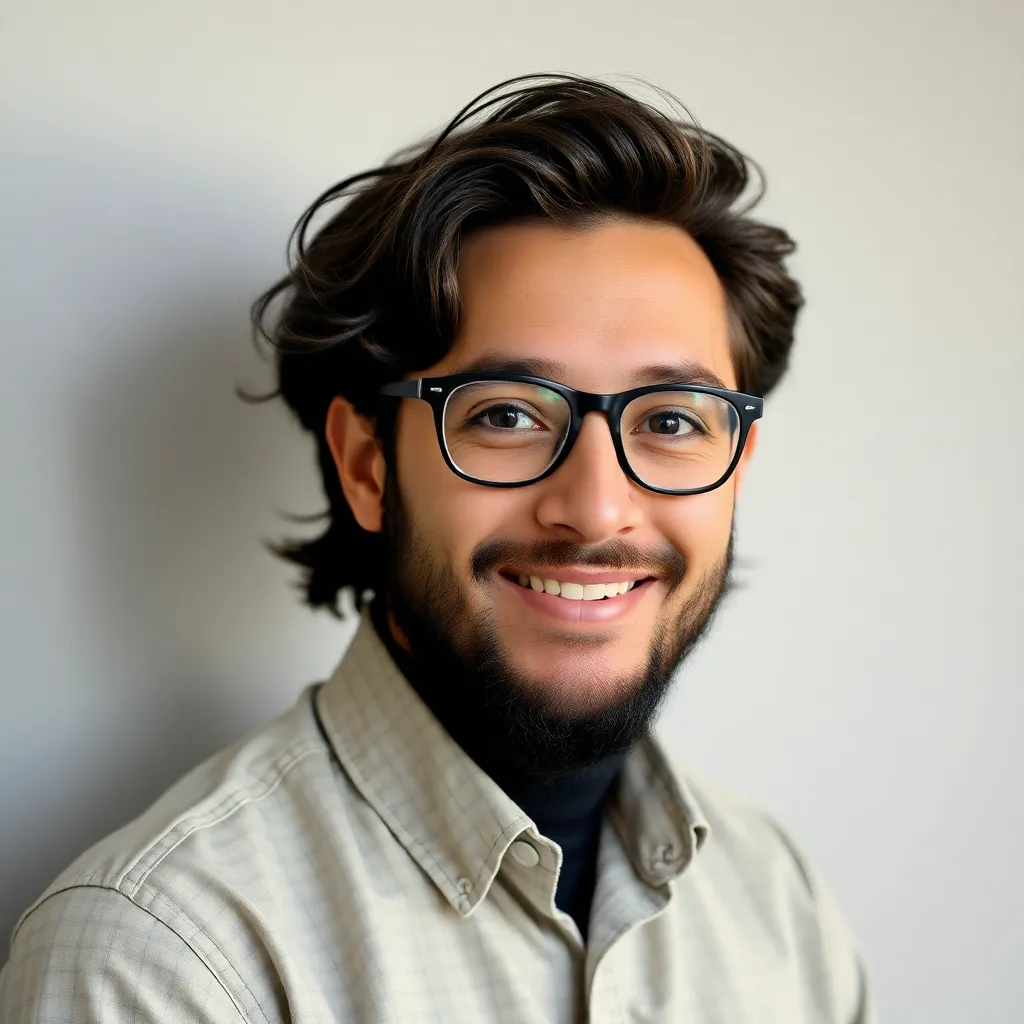
News Co
May 08, 2025 · 6 min read
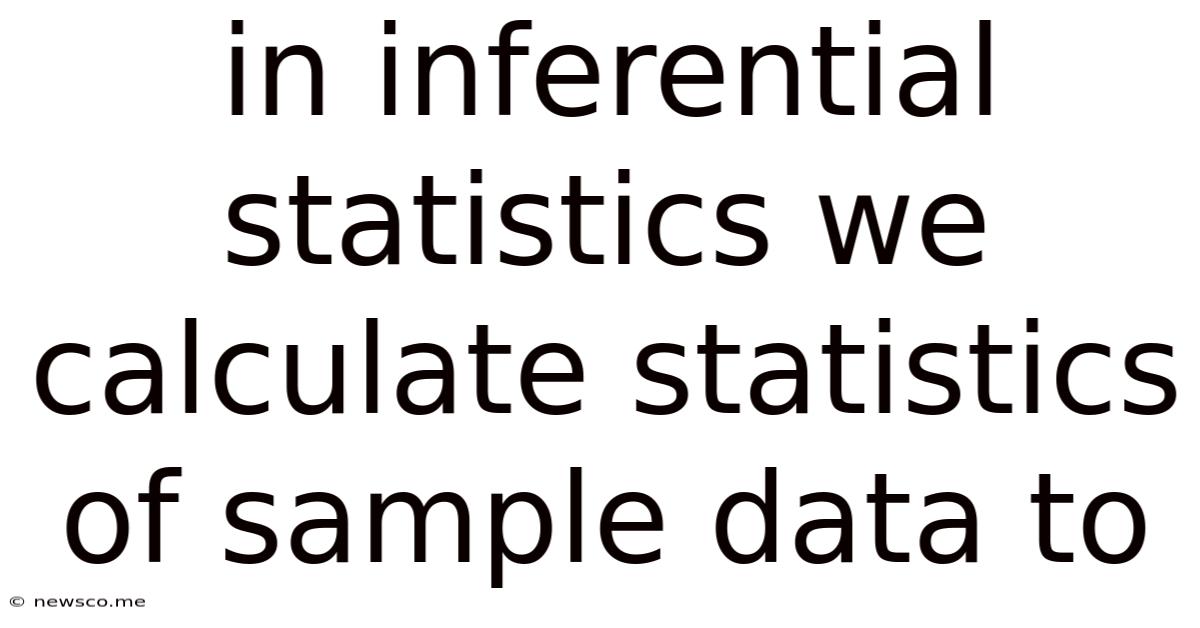
Table of Contents
In Inferential Statistics, We Calculate Statistics of Sample Data To… Make Powerful Inferences About Populations
Inferential statistics is a powerful branch of statistics that allows us to draw conclusions about a population based on data collected from a sample. Instead of examining every single member of a population (which is often impossible or impractical), we analyze a smaller, representative subset and then use statistical methods to generalize our findings to the larger group. This process involves calculating statistics from sample data to make inferences about population parameters. But why do we do this, and how does it work? Let's delve into the core principles and applications.
Understanding the Core Goal: From Sample to Population
The fundamental goal of inferential statistics is to make inferences about population parameters. A population refers to the entire group of individuals, objects, or events that we are interested in studying. For instance, this could be all registered voters in a country, all trees in a specific forest, or all cars manufactured by a particular company in a year. Population parameters are numerical characteristics that describe the population, such as the population mean (average), population standard deviation (spread), or population proportion (percentage). These are often unknown or difficult to obtain directly.
A sample is a smaller subset of the population that is selected for study. It's crucial that the sample is representative of the population; otherwise, our inferences will be biased and unreliable. Various sampling techniques, like simple random sampling, stratified sampling, and cluster sampling, aim to achieve this representativeness. We calculate sample statistics from the sample data – these are estimates of the population parameters.
Key Inferential Statistics Techniques
Several key techniques form the backbone of inferential statistics. These methods leverage the sample statistics to draw conclusions about the population parameters, acknowledging the inherent uncertainty involved in making generalizations from a sample to a population.
1. Estimation
Estimation involves calculating a range of values within which the population parameter is likely to fall. There are two main types of estimation:
-
Point Estimation: This involves calculating a single value as the best estimate of the population parameter. For example, the sample mean is a point estimate of the population mean. While straightforward, it doesn't provide any information about the uncertainty associated with this estimate.
-
Interval Estimation: This approach is far more informative. It creates a confidence interval, which is a range of values that is likely to contain the population parameter with a certain level of confidence. For example, a 95% confidence interval for the population mean implies that if we repeated the sampling process many times, 95% of the calculated confidence intervals would contain the true population mean. The width of the confidence interval reflects the uncertainty – a wider interval indicates greater uncertainty.
2. Hypothesis Testing
Hypothesis testing is a crucial inferential statistical method used to evaluate claims or hypotheses about population parameters. This involves:
-
Formulating Hypotheses: We state two competing hypotheses: a null hypothesis (H₀), which represents the status quo or a default assumption, and an alternative hypothesis (H₁ or Hₐ), which represents the claim we want to investigate.
-
Setting Significance Level (α): This represents the probability of rejecting the null hypothesis when it's actually true (Type I error). A common significance level is 0.05 (5%), meaning there's a 5% chance of concluding there's a difference when there isn't.
-
Collecting and Analyzing Data: We gather sample data and calculate a test statistic, which measures the difference between the sample data and what we would expect under the null hypothesis.
-
Making a Decision: We compare the test statistic to a critical value (determined by the significance level and the distribution of the test statistic). If the test statistic falls within the critical region, we reject the null hypothesis; otherwise, we fail to reject it.
There are various hypothesis testing methods, including:
- t-tests: Used to compare means of two groups.
- z-tests: Used when the population standard deviation is known, or when the sample size is large.
- ANOVA (Analysis of Variance): Used to compare means of three or more groups.
- Chi-square tests: Used to analyze categorical data and test for associations between variables.
3. Regression Analysis
Regression analysis is used to model the relationship between a dependent variable and one or more independent variables. It allows us to predict the value of the dependent variable based on the values of the independent variables. Linear regression is a common type, assuming a linear relationship between variables. Multiple regression allows for multiple independent variables. Regression analysis provides estimates of the relationships between variables, and hypothesis tests can assess the significance of these relationships.
4. Analysis of Variance (ANOVA)
ANOVA is used to test for differences in means across three or more groups. It partitions the total variation in the data into variation between groups and variation within groups. By comparing these variations, ANOVA determines whether the differences between group means are statistically significant. This is particularly useful in experimental designs where we want to compare the effects of different treatments.
Applications of Inferential Statistics
Inferential statistics plays a vital role across numerous fields, including:
-
Healthcare: Analyzing clinical trial data to determine the effectiveness of new drugs or treatments, assessing the prevalence of diseases in populations, and predicting patient outcomes.
-
Business and Finance: Forecasting sales, evaluating marketing campaigns, managing risk, and making investment decisions.
-
Social Sciences: Studying social trends, understanding public opinion, evaluating the effectiveness of social programs, and exploring correlations between social variables.
-
Environmental Science: Analyzing environmental data to assess pollution levels, predict climate change impacts, and monitor ecological changes.
-
Engineering: Assessing the reliability of products, optimizing manufacturing processes, and improving product quality.
Interpreting Results and Avoiding Misinterpretations
Interpreting the results of inferential statistical analyses requires careful consideration. Here's what to keep in mind:
-
Statistical Significance vs. Practical Significance: A statistically significant result indicates that the observed effect is unlikely to be due to chance alone. However, it doesn't necessarily mean the effect is practically significant or meaningful in the real world. The magnitude of the effect and its practical implications should always be evaluated.
-
Sample Size: The sample size significantly impacts the reliability of inferential statistics. Larger samples generally lead to more precise estimates and greater power in hypothesis testing.
-
Sampling Bias: Bias in the sampling method can lead to inaccurate inferences about the population. It's crucial to employ appropriate sampling techniques to minimize bias.
-
Causation vs. Correlation: Inferential statistics can reveal correlations between variables, but correlation does not imply causation. Observing a relationship between two variables doesn't necessarily mean that one causes the other. Further investigation is needed to establish causal relationships.
-
Limitations of the Model: Statistical models are simplifications of reality. They may not perfectly capture all the complexities of the phenomenon under study. It's essential to acknowledge the limitations of the model and its assumptions.
Conclusion: The Power and Responsibility of Inference
Inferential statistics provides a powerful set of tools for drawing conclusions about populations based on sample data. It's essential in making evidence-based decisions across numerous fields. However, responsible application requires careful attention to the assumptions, limitations, and potential for misinterpretations. By understanding the core principles and techniques, researchers and practitioners can harness the power of inferential statistics to gain valuable insights and make informed decisions based on data. Remember, the process is not just about calculating statistics; it's about using those statistics to build a sound understanding of the world around us. The ultimate goal is to use sample data to accurately reflect the characteristics of the population, leading to meaningful and reliable conclusions. This careful and nuanced approach ensures the responsible and effective use of this powerful branch of statistics.
Latest Posts
Latest Posts
-
Cuanto Es 16 Grados Fahrenheit En Centigrados
May 08, 2025
-
Which Of The Following Is An Algebraic Expression
May 08, 2025
-
How Much Is 6000 Lb In American Money
May 08, 2025
-
A Line Segment May Have More Than One Midpoint
May 08, 2025
-
Write 84 As A Product Of Prime Factors
May 08, 2025
Related Post
Thank you for visiting our website which covers about In Inferential Statistics We Calculate Statistics Of Sample Data To . We hope the information provided has been useful to you. Feel free to contact us if you have any questions or need further assistance. See you next time and don't miss to bookmark.