Probability Distribution Mean And Standard Deviation
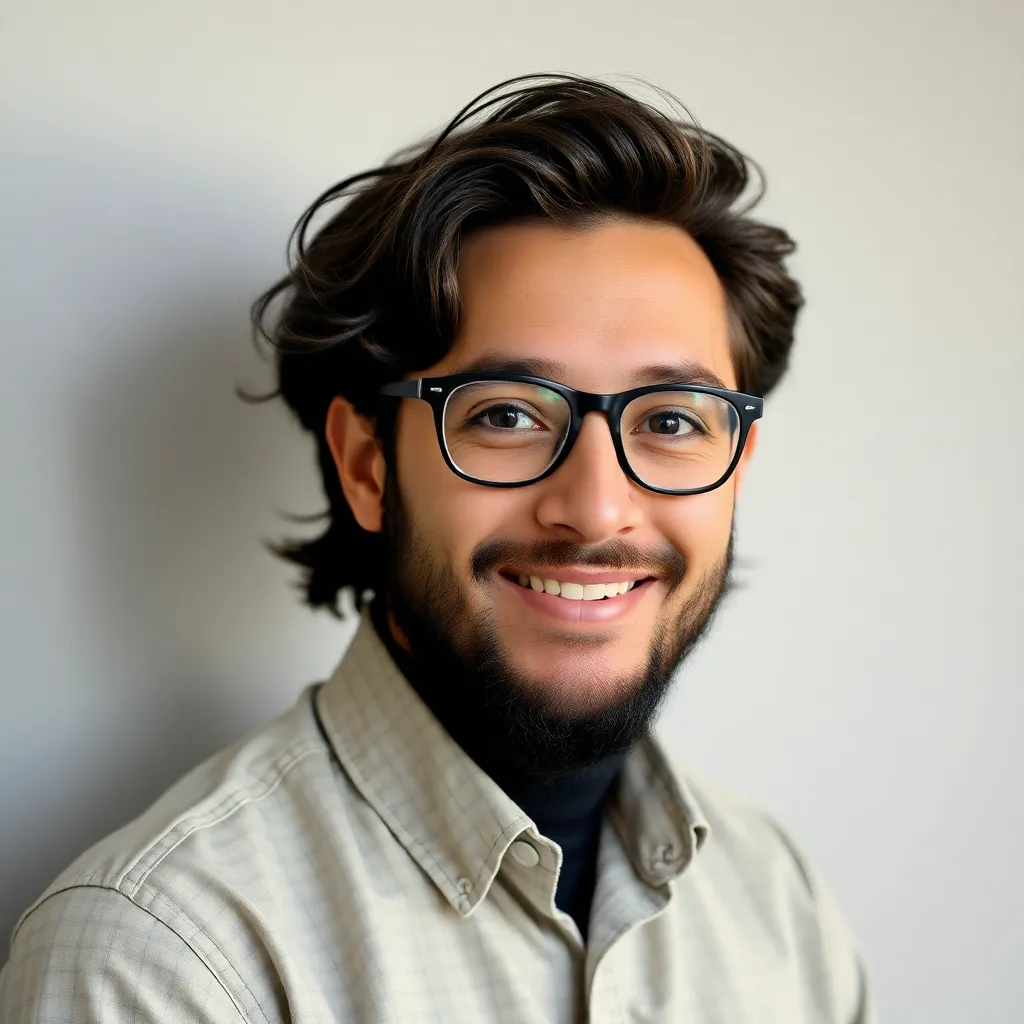
News Co
May 03, 2025 · 6 min read
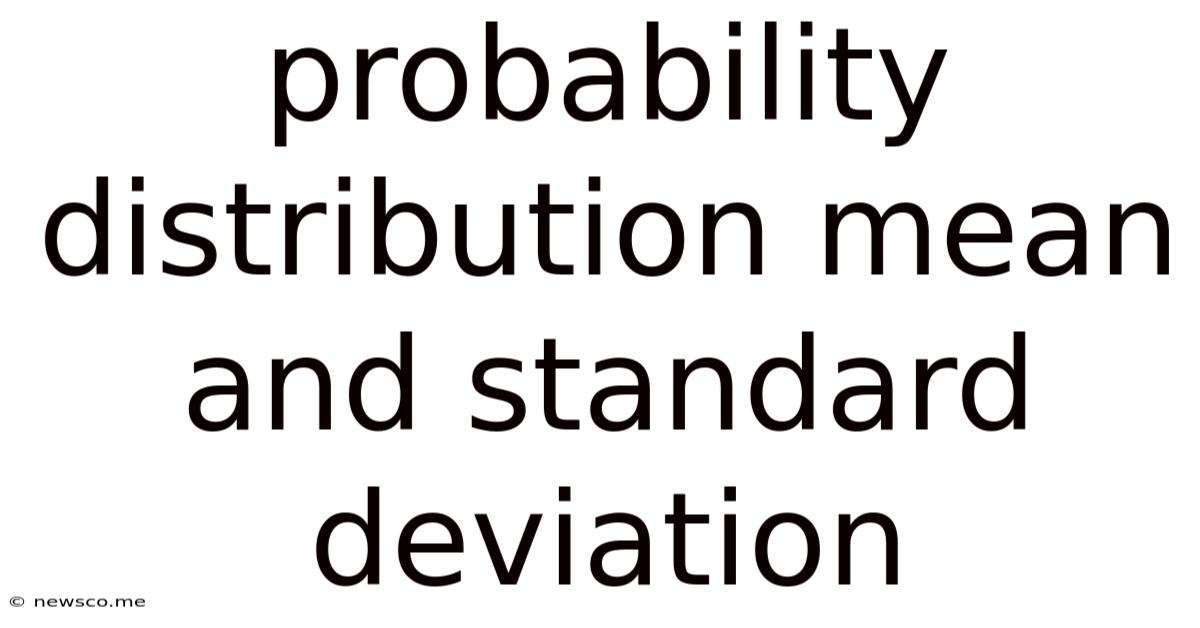
Table of Contents
Probability Distribution: Mean and Standard Deviation – A Deep Dive
Understanding probability distributions is crucial in numerous fields, from finance and engineering to medicine and social sciences. This comprehensive guide delves into the core concepts of mean and standard deviation within the context of probability distributions, providing a detailed explanation accessible to both beginners and those seeking a deeper understanding. We'll explore various distribution types and demonstrate how these key parameters describe the central tendency and spread of data.
What is a Probability Distribution?
A probability distribution is a mathematical function that provides the probabilities of occurrence of different possible outcomes for a random variable. It essentially describes the likelihood of different values a variable can take. Think of it as a summary of all potential outcomes and their associated chances. For example, the probability distribution for the roll of a fair six-sided die would assign a probability of 1/6 to each outcome (1, 2, 3, 4, 5, 6).
There are two main types of probability distributions:
-
Discrete Probability Distributions: These deal with discrete variables – variables that can only take on specific, separate values (like the number of heads when flipping a coin three times). Examples include the binomial distribution, Poisson distribution, and geometric distribution.
-
Continuous Probability Distributions: These handle continuous variables – variables that can take on any value within a given range (like the height of a student). Examples include the normal distribution, exponential distribution, and uniform distribution.
Mean: The Measure of Central Tendency
The mean, often referred to as the average, is a measure of the central tendency of a probability distribution. It represents the expected value of the random variable. In simpler terms, it indicates where the "center" of the distribution lies.
Calculating the Mean:
The calculation of the mean differs slightly depending on whether the distribution is discrete or continuous:
Discrete Distributions: The mean (μ) is calculated as the weighted average of all possible outcomes, where the weights are the probabilities of each outcome:
μ = Σ [x * P(x)]
Where:
- x represents each possible outcome.
- P(x) represents the probability of outcome x.
- Σ denotes the sum over all possible outcomes.
Continuous Distributions: For continuous distributions, the mean is calculated using an integral:
μ = ∫ x * f(x) dx
Where:
- x represents the value of the continuous variable.
- f(x) represents the probability density function (PDF) of the distribution.
- ∫ denotes the integral over the entire range of x.
Mean's Significance:
The mean is a vital statistic because it provides a concise summary of the distribution's central location. It’s frequently used for comparison between different distributions or datasets. However, it’s important to note that the mean can be significantly influenced by outliers (extreme values) in the data.
Standard Deviation: The Measure of Spread
The standard deviation (σ) measures the dispersion or spread of a probability distribution. It quantifies how much the data points deviate from the mean. A larger standard deviation indicates greater variability, while a smaller standard deviation indicates data points are clustered closely around the mean.
Calculating the Standard Deviation:
Similar to the mean, the calculation of the standard deviation differs slightly for discrete and continuous distributions:
Discrete Distributions: The standard deviation is calculated as the square root of the variance (σ²):
σ = √[Σ [(x - μ)² * P(x)]]
Where:
- x represents each possible outcome.
- μ represents the mean of the distribution.
- P(x) represents the probability of outcome x.
- Σ denotes the sum over all possible outcomes.
Continuous Distributions: For continuous distributions, the standard deviation is calculated as the square root of the variance, which is found using an integral:
σ = √[∫ (x - μ)² * f(x) dx]
Where:
- x represents the value of the continuous variable.
- μ represents the mean of the distribution.
- f(x) represents the probability density function (PDF) of the distribution.
- ∫ denotes the integral over the entire range of x.
Standard Deviation's Significance:
The standard deviation provides crucial information about the data's variability. It's often used in conjunction with the mean to characterize a dataset completely. For instance, knowing both the mean and standard deviation of exam scores allows you to understand not only the average performance but also the spread of scores, indicating the consistency of performance within the group.
A high standard deviation suggests a wide range of scores, indicating significant variability, while a low standard deviation implies scores are tightly clustered around the average.
Illustrative Examples: Common Probability Distributions
Let's explore the mean and standard deviation for a few common probability distributions:
1. Normal Distribution:
The normal distribution, also known as the Gaussian distribution, is arguably the most important probability distribution in statistics. It’s characterized by its bell-shaped curve, symmetrical around the mean.
- Mean (μ): The mean is located at the center of the bell curve.
- Standard Deviation (σ): It determines the width of the bell curve. A larger σ leads to a wider, flatter curve, while a smaller σ results in a narrower, taller curve. Approximately 68% of the data falls within one standard deviation of the mean, 95% within two standard deviations, and 99.7% within three standard deviations.
2. Binomial Distribution:
The binomial distribution models the probability of a certain number of successes in a fixed number of independent Bernoulli trials (trials with only two possible outcomes, success or failure).
- Mean (μ): μ = n * p, where 'n' is the number of trials and 'p' is the probability of success in a single trial.
- Standard Deviation (σ): σ = √[n * p * (1 - p)]
3. Poisson Distribution:
The Poisson distribution describes the probability of a given number of events occurring in a fixed interval of time or space if these events occur with a known average rate and independently of the time since the last event.
- Mean (μ): μ = λ (lambda), which represents the average rate of events.
- Standard Deviation (σ): σ = √λ
4. Uniform Distribution:
The uniform distribution assigns equal probability to all outcomes within a specified range.
- Mean (μ): μ = (a + b) / 2, where 'a' and 'b' are the lower and upper bounds of the range.
- Standard Deviation (σ): σ = (b - a) / √12
Applying Mean and Standard Deviation: Real-World Applications
Understanding mean and standard deviation has far-reaching applications across various disciplines:
- Finance: Analyzing stock returns, calculating portfolio risk, and assessing investment performance.
- Healthcare: Evaluating the effectiveness of treatments, studying disease prevalence, and managing patient risk.
- Engineering: Quality control, process optimization, and reliability analysis.
- Social Sciences: Analyzing survey data, studying population trends, and understanding social behaviors.
- Climate Science: Analyzing temperature data, predicting weather patterns, and assessing climate change impacts.
Conclusion: The Power of Understanding Probability Distributions
The mean and standard deviation are fundamental parameters that provide a powerful way to summarize and understand probability distributions. They offer insights into the central tendency and variability of data, enabling effective analysis and informed decision-making across numerous fields. Mastering these concepts is essential for anyone working with data analysis, statistical modeling, or any field where understanding uncertainty is critical. By understanding the nuances of these measures within different distribution types, you can unlock a deeper understanding of the data you're working with and make more accurate predictions and inferences. Further exploration into more advanced statistical concepts will build upon this foundation, allowing you to tackle increasingly complex problems.
Latest Posts
Latest Posts
-
Ratio Of The Circumference Of A Circle To Its Diameter
May 07, 2025
-
Square Root Of 40 Simplified Radical Form
May 07, 2025
-
Where Is 1 3 On The Number Line
May 07, 2025
-
What Is 40 Off Of 80 Dollars
May 07, 2025
-
How To Write 450 On A Check
May 07, 2025
Related Post
Thank you for visiting our website which covers about Probability Distribution Mean And Standard Deviation . We hope the information provided has been useful to you. Feel free to contact us if you have any questions or need further assistance. See you next time and don't miss to bookmark.