Relative Frequencies Are Calculated As The:
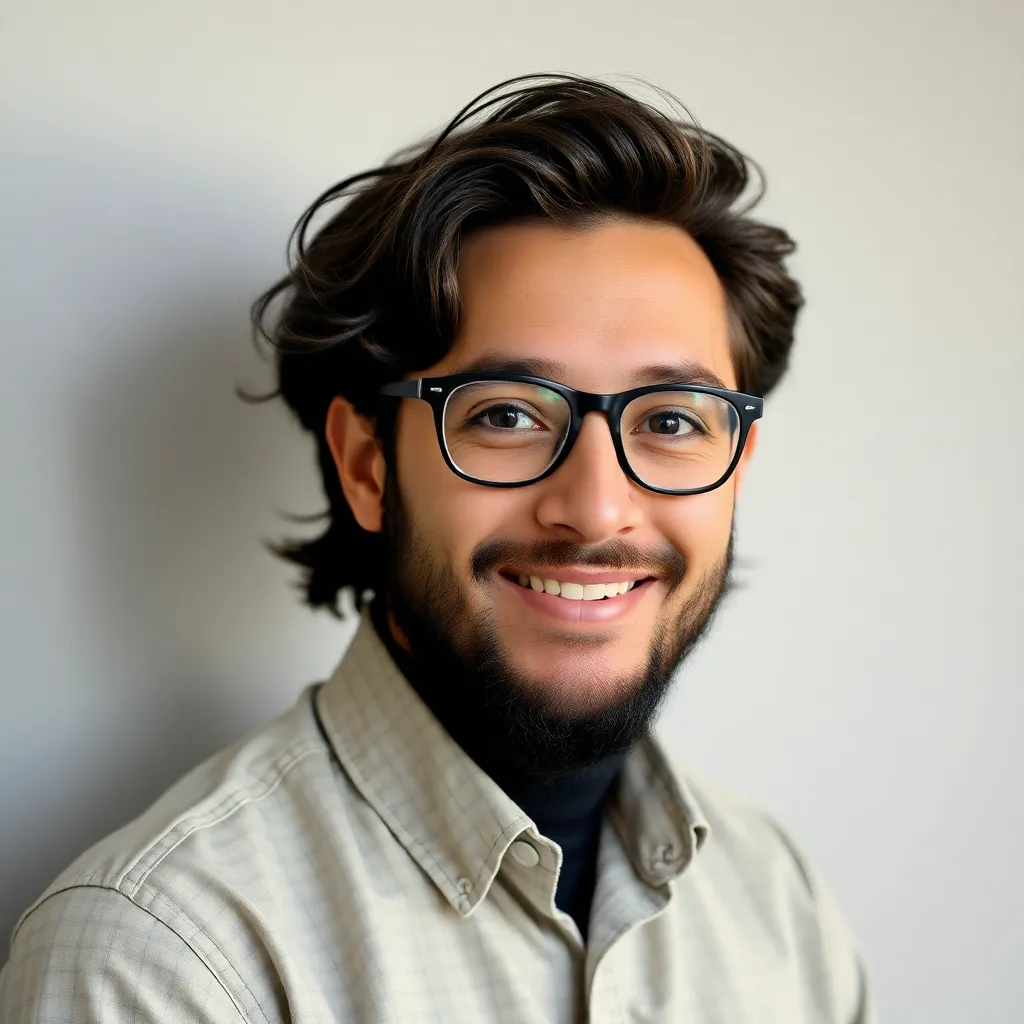
News Co
May 04, 2025 · 6 min read

Table of Contents
Relative Frequencies: A Deep Dive into Calculation and Application
Relative frequency, a cornerstone concept in statistics and data analysis, represents the proportion or percentage of times a specific event or value occurs within a dataset. Unlike absolute frequencies, which simply count occurrences, relative frequencies provide a normalized perspective, allowing for easier comparison across datasets of varying sizes and facilitating a deeper understanding of data distribution. This comprehensive guide delves into the intricacies of calculating relative frequencies, exploring diverse applications, and highlighting its significance in various fields.
Understanding the Fundamentals: Absolute vs. Relative Frequency
Before delving into the calculation of relative frequencies, it's crucial to grasp the distinction between absolute and relative frequencies.
Absolute Frequency: This refers to the raw count of the number of times a particular event or value appears in a dataset. For instance, if we surveyed 100 people about their favorite color, and 25 responded with "blue," the absolute frequency of "blue" is 25.
Relative Frequency: This represents the proportion of times a specific event occurs relative to the total number of events. It's calculated by dividing the absolute frequency of an event by the total number of events. In our color survey example, the relative frequency of "blue" would be 25/100 = 0.25 or 25%. This indicates that 25% of the respondents favored blue.
This normalization is critical because it allows for direct comparison between datasets with different sizes. For example, if another survey of 200 people showed 60 people preferred blue, the absolute frequency is higher (60 > 25), but the relative frequency (60/200 = 0.30 or 30%) reveals that a larger proportion of respondents in the second survey chose blue.
Calculating Relative Frequencies: Step-by-Step Guide
Calculating relative frequencies is a straightforward process, typically involving these steps:
-
Gather and Organize Data: Begin by collecting and organizing your data. This may involve creating a frequency table, a histogram, or other suitable data representation depending on the nature of your data. The key is to have a clear count of each event or value.
-
Calculate Absolute Frequencies: Count the occurrences of each unique event or value in your dataset. This gives you the absolute frequency for each category.
-
Determine the Total Number of Observations: Sum up all the absolute frequencies to get the total number of observations (N) in your dataset.
-
Calculate Relative Frequencies: For each event or value, divide its absolute frequency by the total number of observations (N). This gives you the relative frequency for that specific event. The formula is:
Relative Frequency = (Absolute Frequency) / (Total Number of Observations)
-
Express as Percentage (Optional): Multiply the relative frequency by 100 to express it as a percentage. This provides a more easily interpretable representation for many applications.
Example:
Let's consider the following data representing the number of cars of different colors observed in a parking lot:
Car Color | Absolute Frequency |
---|---|
Red | 15 |
Blue | 10 |
Green | 5 |
White | 20 |
Total | 50 |
To calculate the relative frequencies:
- Relative Frequency (Red): 15/50 = 0.3 or 30%
- Relative Frequency (Blue): 10/50 = 0.2 or 20%
- Relative Frequency (Green): 5/50 = 0.1 or 10%
- Relative Frequency (White): 20/50 = 0.4 or 40%
This clearly shows that white cars constitute the largest proportion (40%) in the parking lot, followed by red cars (30%).
Applications of Relative Frequencies: Unveiling Insights Across Disciplines
Relative frequencies are a powerful tool with broad applicability across numerous fields. Here are some prominent examples:
1. Market Research and Business Analytics:
Relative frequencies are indispensable in analyzing market trends and consumer behavior. For example, understanding the relative frequency of different product purchases helps businesses optimize inventory, tailor marketing strategies, and identify emerging trends. Analyzing customer demographics using relative frequencies provides valuable insights for targeted advertising.
2. Scientific Research and Experimental Design:
In scientific research, relative frequencies are crucial for analyzing experimental outcomes. For instance, in drug trials, the relative frequency of positive responses in the treatment group compared to the control group is critical in determining the effectiveness of a new drug. Similarly, studies in ecology use relative frequencies to analyze species distribution and population dynamics.
3. Quality Control and Manufacturing:
Manufacturing processes often utilize relative frequency analysis to assess defect rates. By tracking the relative frequency of defective products, manufacturers can identify bottlenecks in their production lines and implement corrective measures to improve quality control.
4. Healthcare and Epidemiology:
Relative frequencies play a critical role in epidemiology, aiding in the understanding of disease prevalence and incidence rates. Analyzing the relative frequencies of different diseases within a population helps public health officials allocate resources effectively and develop targeted prevention programs.
5. Social Sciences and Demography:
Relative frequencies are invaluable tools in social sciences and demographic studies. For instance, analyzing relative frequencies of different ethnic groups within a population helps researchers understand social dynamics and potential disparities. Similarly, studying the relative frequencies of different income levels provides insights into economic inequality.
6. Probability and Statistics:
At the heart of probability theory, relative frequencies form the foundation for estimating probabilities. In large samples, the relative frequency of an event often serves as a good approximation of its probability. This concept is crucial in statistical inference and hypothesis testing.
Interpreting and Visualizing Relative Frequencies: Communicating Data Effectively
Once calculated, relative frequencies need to be effectively interpreted and communicated. This often involves using appropriate visualization techniques.
-
Bar Charts: A straightforward way to visualize relative frequencies, bar charts represent each category with a bar whose length corresponds to its relative frequency. This makes it easy to compare the proportions of different categories.
-
Pie Charts: Pie charts are particularly useful for displaying the relative frequencies of different categories as proportions of a whole. Each slice of the pie represents a category, and its size is proportional to its relative frequency.
-
Histograms: When dealing with continuous data, histograms display relative frequencies using bars representing ranges of values. The height of each bar reflects the relative frequency of data points falling within that specific range.
-
Frequency Polygons: Similar to histograms, frequency polygons use lines to connect points representing the midpoints of each class interval. They provide a visual representation of the distribution's shape and can show trends more clearly than histograms.
Beyond Basic Calculation: Advanced Applications and Considerations
While calculating basic relative frequencies is straightforward, advanced applications involve more sophisticated techniques:
-
Cumulative Relative Frequencies: These represent the accumulated relative frequency up to a certain point in a data set. This is useful for determining the percentage of observations falling below a certain value.
-
Conditional Relative Frequencies: These represent the relative frequency of an event given that another event has already occurred. This is often used in analyzing relationships between variables.
-
Joint Relative Frequencies: When dealing with multiple variables, joint relative frequencies represent the proportion of observations with specific combinations of values. This is helpful in examining correlations and interactions between variables.
Conclusion: The Power of Relative Frequencies in Data Analysis
Relative frequencies offer a versatile and powerful tool for analyzing and interpreting data. By normalizing absolute frequencies, they enable comparisons across datasets of different sizes and provide valuable insights into data distribution. Their application spans diverse fields, making them an indispensable technique in various analytical endeavors. Understanding the calculation and interpretation of relative frequencies empowers individuals and organizations to make more informed decisions based on data-driven insights. Mastering this fundamental statistical concept opens doors to a deeper understanding of data and its implications in numerous contexts.
Latest Posts
Latest Posts
-
At What Point Does The Curve Have Maximum Curvature
May 05, 2025
-
3 X 2 4 X 2
May 05, 2025
-
Name The Quadrant Or Axis Where Each Point Lies
May 05, 2025
-
Counting By 3 Chart To 100
May 05, 2025
-
41 8 As A Mixed Number
May 05, 2025
Related Post
Thank you for visiting our website which covers about Relative Frequencies Are Calculated As The: . We hope the information provided has been useful to you. Feel free to contact us if you have any questions or need further assistance. See you next time and don't miss to bookmark.