The Median Is The Same As The Quartile
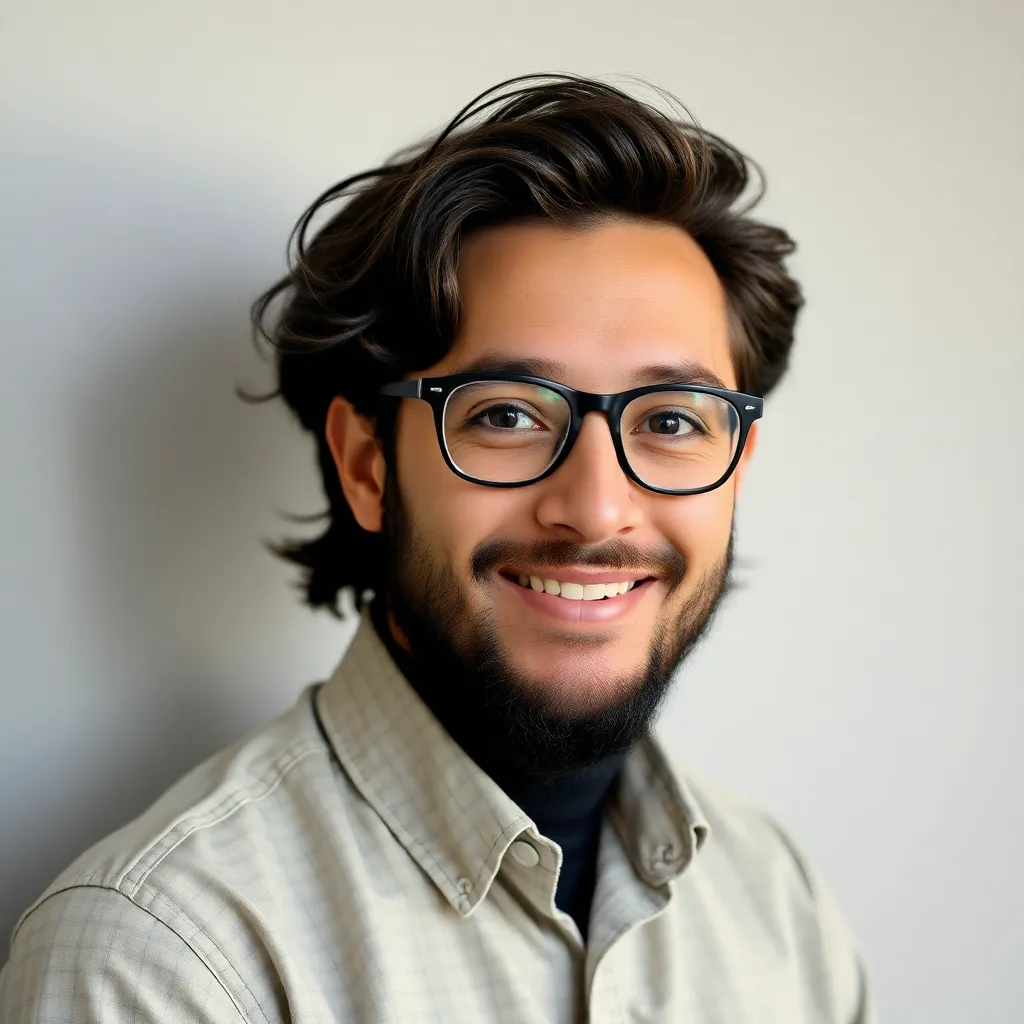
News Co
May 07, 2025 · 6 min read
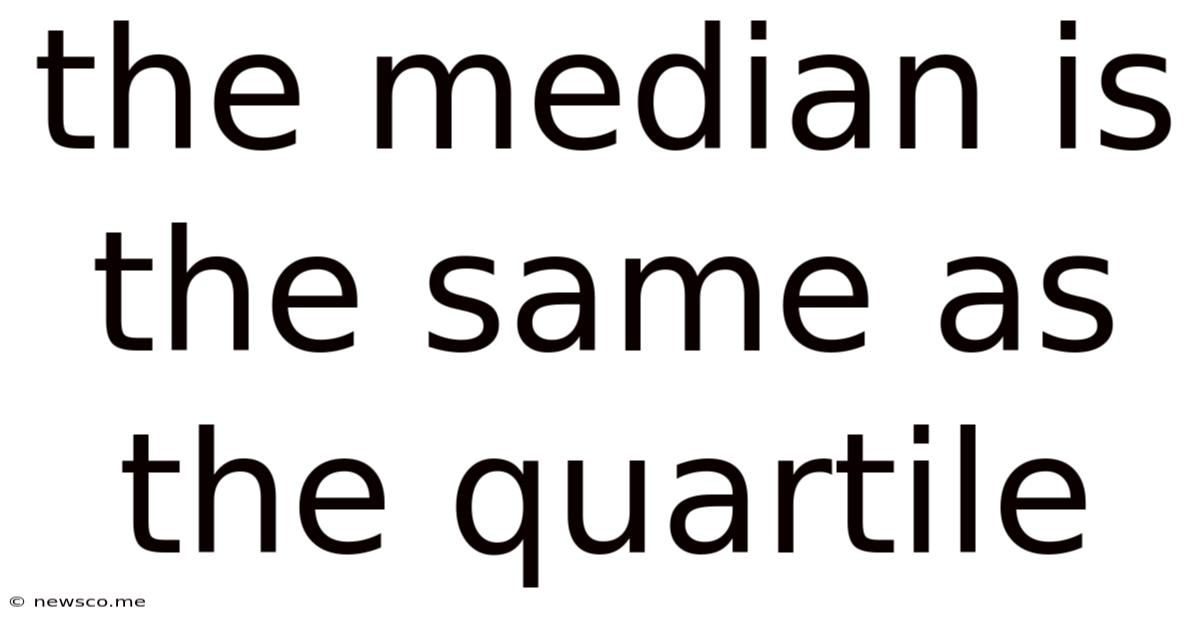
Table of Contents
The Median: More Than Just the Middle Ground – Understanding its Relationship with Quartiles
The median, a fundamental concept in statistics, often gets simplified to "the middle value." While this is true for datasets with an odd number of observations, a more nuanced understanding reveals its deeper connection to the quartiles and its crucial role in descriptive statistics. This article delves into the intricate relationship between the median and quartiles, clarifying misconceptions and highlighting its importance in data analysis. We'll explore how the median acts as the second quartile, delve into the calculation methods for both with various data types, and discuss its implications in data interpretation and visualization.
Understanding the Median: The Heart of the Distribution
The median represents the central point of a dataset when it's ordered numerically. It divides the data into two equal halves: 50% of the data points fall below the median, and 50% fall above it. This makes it a robust measure of central tendency, less susceptible to outliers than the mean (average).
Calculating the Median:
-
Odd Number of Observations: When the dataset has an odd number of values, the median is simply the middle value. For example, in the dataset {2, 4, 6, 8, 10}, the median is 6.
-
Even Number of Observations: When the dataset has an even number of values, the median is the average of the two middle values. For example, in the dataset {2, 4, 6, 8}, the median is (4 + 6) / 2 = 5.
Decoding Quartiles: Dividing Data into Fourths
Quartiles, as the name suggests, divide an ordered dataset into four equal parts. We have three key quartiles:
-
First Quartile (Q1): This separates the bottom 25% of the data from the top 75%. It's also known as the lower quartile.
-
Second Quartile (Q2): This separates the bottom 50% of the data from the top 50%. Guess what? This is the median!
-
Third Quartile (Q3): This separates the bottom 75% of the data from the top 25%. It's also known as the upper quartile.
The Inseparable Link: Median as the Second Quartile
The crucial connection to understand is that the median is identical to the second quartile (Q2). This isn't just a coincidence; it's a direct consequence of how both are defined. Both values represent the point where 50% of the data lies below and 50% lies above.
Calculating Quartiles: Methods and Considerations
Calculating quartiles, especially for larger datasets, often involves interpolation techniques. Let's look at the most common approaches:
Method 1: Using the Position Formula
This method utilizes a formula to determine the position of each quartile within the ordered dataset. The formula for the position of the kth quartile (Q<sub>k</sub>) is:
Position = k * (n + 1) / 4
Where:
k
= the quartile number (1, 2, or 3)n
= the number of observations in the dataset
For example, with a dataset of 10 values, the position of Q1 would be: 1 * (10 + 1) / 4 = 2.75. Since this is not a whole number, we interpolate between the 2nd and 3rd values.
Method 2: Linear Interpolation
When the position calculated using the formula above is not a whole number, linear interpolation is used to estimate the quartile value. This involves calculating a weighted average of the two surrounding values.
For instance, if the position of Q1 is 2.75, and the 2nd and 3rd values are 5 and 7, respectively, the interpolated Q1 would be:
Q1 = 0.75 * 7 + 0.25 * 5 = 6.5
Method 3: Software and Statistical Packages
Statistical software packages like R, SPSS, and Excel provide built-in functions for calculating quartiles. These programs usually employ sophisticated algorithms that handle various scenarios and data distributions accurately. It's recommended to use these tools for larger datasets to ensure precise and efficient calculations.
The Significance of Median and Quartiles in Data Analysis
The median and quartiles are essential tools for understanding the distribution and characteristics of a dataset. They are particularly valuable when:
-
Outliers are present: The median is far less affected by extreme values than the mean, providing a more robust measure of central tendency.
-
Skewness is detected: The relationship between the median, mean, and quartiles reveals the skewness of the distribution. A skewed distribution will have a different distance between the median and Q1 compared to the distance between the median and Q3.
-
Descriptive statistics are needed: The median and quartiles provide a concise summary of the data's spread and central tendency. They are often used in conjunction with the minimum and maximum values to create box plots, which offer a visual representation of the data distribution.
Box Plots: Visualizing Data with Median and Quartiles
Box plots are powerful visual tools that utilize the median and quartiles to represent the distribution of data. Key elements of a box plot include:
-
The box: Represents the interquartile range (IQR), which is the difference between Q3 and Q1 (IQR = Q3 - Q1). The median is marked within the box.
-
Whiskers: Extend from the box to the minimum and maximum values within a certain range (usually 1.5 * IQR from the quartiles).
-
Outliers: Data points outside the whisker range are plotted as individual points, highlighting extreme values.
Box plots offer a quick and efficient way to compare the distributions of multiple datasets or to identify outliers.
Handling Different Data Types: Continuous vs. Discrete
While the principles remain the same, the calculation approach might vary slightly depending on whether your data is continuous (e.g., height, weight) or discrete (e.g., number of cars, number of children). For discrete data, interpolation might be less straightforward, and sometimes the quartile positions might fall directly on a data point. In these cases, the quartile is simply the data point at that position.
Applications in Real-World Scenarios
The importance of the median and quartiles extends across various fields:
-
Finance: Analyzing stock prices, income distributions, and risk assessments.
-
Healthcare: Studying patient recovery times, disease prevalence, and treatment effectiveness.
-
Education: Evaluating student test scores, comparing academic performance, and identifying areas for improvement.
-
Engineering: Quality control, assessing product reliability, and process optimization.
-
Environmental Science: Monitoring pollution levels, analyzing climate change data, and understanding ecological trends.
Conclusion: A Deeper Appreciation for the Median's Role
The median, though seemingly simple, plays a multifaceted role in statistical analysis. Its identity as the second quartile underscores its importance in understanding data distribution, especially when dealing with skewed data or the presence of outliers. Mastering the calculation and interpretation of both the median and quartiles enhances your ability to extract valuable insights from your data and communicate your findings effectively. Using these concepts in conjunction with visualization tools like box plots allows for a more comprehensive understanding of your dataset and strengthens your ability to make data-driven decisions. Remember to leverage statistical software for accurate calculations, especially when dealing with large datasets. A deeper appreciation for the median's role and its close relationship with quartiles equips you with invaluable tools for data analysis in any field.
Latest Posts
Related Post
Thank you for visiting our website which covers about The Median Is The Same As The Quartile . We hope the information provided has been useful to you. Feel free to contact us if you have any questions or need further assistance. See you next time and don't miss to bookmark.