The Square Of The Standard Deviation Is Called The
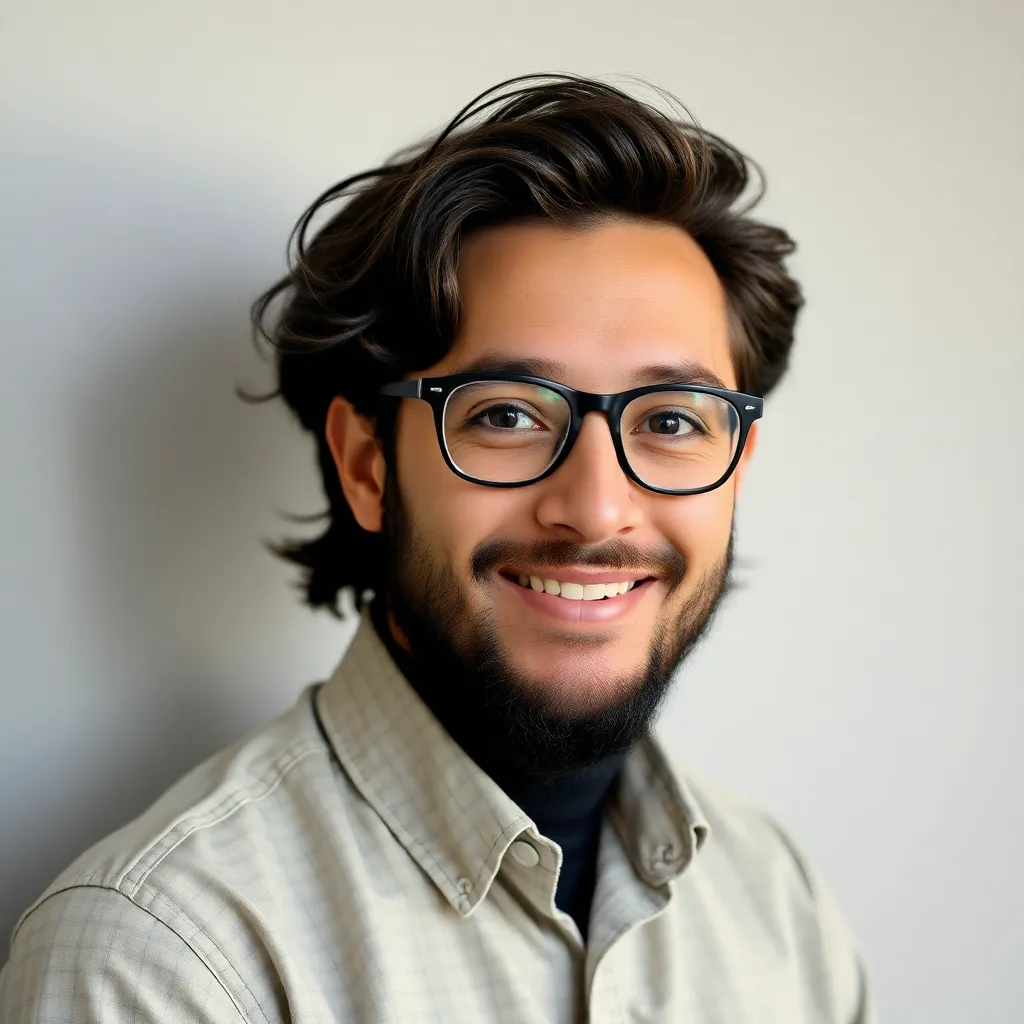
News Co
Mar 08, 2025 · 7 min read

Table of Contents
The Square of the Standard Deviation is Called the Variance: A Deep Dive into Statistical Dispersion
The square of the standard deviation is called the variance. Understanding variance is crucial in statistics because it quantifies the spread or dispersion of a dataset around its mean. While the standard deviation provides a readily interpretable measure of spread in the original units of the data, the variance offers a mathematically convenient measure that's fundamental to many statistical analyses and models. This article will delve deep into the concept of variance, exploring its calculation, interpretation, and applications. We'll also examine its relationship with the standard deviation and other related statistical measures.
Understanding Variance: A Measure of Dispersion
In simple terms, variance measures how far a set of numbers is spread out from their average value. A high variance indicates that the data points are far from the mean, implying high variability. Conversely, a low variance suggests that the data points cluster closely around the mean, indicating low variability.
Imagine two datasets:
- Dataset A: 10, 12, 11, 13, 10
- Dataset B: 5, 15, 10, 20, 0
Both datasets have a mean of 11.2. However, Dataset B displays a much wider spread of values than Dataset A. This difference in spread is captured by the variance. Dataset B will have a significantly higher variance than Dataset A.
Why Use Variance?
While the standard deviation is often preferred for its interpretability (as it's in the same units as the data), variance holds significant advantages:
- Mathematical Properties: Variance possesses desirable mathematical properties that make it easier to work with in more advanced statistical techniques. For example, variances of independent random variables can be added directly, a property that's not true for standard deviations. This is crucial in areas like ANOVA (Analysis of Variance) and regression analysis.
- Underlying Calculations: Many statistical tests and models rely on the calculation of variance as a foundational step. Understanding variance provides a deeper understanding of these models.
- Sensitivity to Outliers: Both variance and standard deviation are sensitive to outliers (extreme values). This sensitivity highlights the importance of examining the data for outliers before drawing conclusions based on these measures.
Calculating Variance: A Step-by-Step Guide
The calculation of variance involves several steps:
-
Calculate the Mean (Average): Find the average of the data set. This is done by summing all the values and dividing by the number of values (n).
-
Find the Deviations from the Mean: For each data point, subtract the mean from its value. This gives you the deviation of each data point from the average.
-
Square the Deviations: Square each of the deviations calculated in step 2. This is crucial because squaring eliminates negative values, ensuring that all deviations contribute positively to the overall measure of spread.
-
Sum of Squared Deviations: Add up all the squared deviations from step 3. This sum represents the total squared deviation from the mean.
-
Divide by n-1 (Sample Variance) or n (Population Variance): This is the final step. The divisor used depends on whether you're working with a sample or the entire population.
-
Sample Variance: When dealing with a sample (a subset of the population), divide the sum of squared deviations by (n-1), where 'n' is the sample size. This is known as Bessel's correction and it helps to provide an unbiased estimate of the population variance.
-
Population Variance: If you have data for the entire population, divide the sum of squared deviations by 'n', the population size.
-
Formulae:
-
Population Variance (σ²): σ² = Σ(xᵢ - μ)² / n
- Where:
- σ² is the population variance
- xᵢ represents each individual data point
- μ is the population mean
- n is the population size
- Σ denotes the summation
- Where:
-
Sample Variance (s²): s² = Σ(xᵢ - x̄)² / (n-1)
- Where:
- s² is the sample variance
- xᵢ represents each individual data point
- x̄ is the sample mean
- n is the sample size
- Σ denotes the summation
- Where:
The sample variance (s²) is an estimator of the population variance (σ²). Using (n-1) instead of n in the sample variance calculation provides a less biased estimate of the population variance.
Variance vs. Standard Deviation: Understanding the Difference
The standard deviation (σ or s) is simply the square root of the variance (σ² or s²). While both measure dispersion, they differ in their units and interpretation:
-
Units: Variance is measured in squared units of the original data, making it less intuitive to interpret directly. For example, if the data represents heights in centimeters, the variance is in square centimeters. The standard deviation, on the other hand, is in the same units as the original data (centimeters in this case), making it easier to understand in the context of the data.
-
Interpretation: The standard deviation represents the average distance of data points from the mean. A larger standard deviation indicates greater variability, while a smaller standard deviation indicates less variability. This is more readily understandable than interpreting the variance directly.
Essentially, the standard deviation is the more practical measure for directly interpreting the spread of data, whereas the variance is crucial for many statistical calculations and theoretical underpinnings.
Applications of Variance
Variance plays a pivotal role in various statistical applications, including:
-
Hypothesis Testing: Many hypothesis tests, like the t-test and ANOVA, rely on variance calculations to determine the statistical significance of results. These tests use variances to compare the means of different groups or to assess the effect of independent variables.
-
Regression Analysis: In regression analysis, variance is used to measure the goodness of fit of a model. The variance explained by the model is compared to the total variance in the data to determine how well the model predicts the dependent variable.
-
Portfolio Management (Finance): In finance, variance (and its related measure, standard deviation) is used as a measure of risk. A higher variance in portfolio returns implies higher risk.
-
Quality Control: In manufacturing and quality control, variance is used to monitor the consistency of a production process. Low variance indicates a more consistent and reliable process.
-
Machine Learning: Variance is a critical concept in machine learning, especially in areas like model evaluation and feature selection. High variance in a model can indicate overfitting, where the model performs well on training data but poorly on unseen data.
Interpreting Variance Values
The magnitude of the variance itself doesn't provide a standardized interpretation across all datasets. It depends heavily on the scale and nature of the data. However, a higher variance relative to another dataset implies greater variability. The most useful interpretation of variance comes through its relationship with the standard deviation and how it contributes to the understanding of data dispersion and variability within a particular context.
Limitations of Variance
While variance is a powerful statistical tool, it has limitations:
-
Sensitivity to Outliers: As mentioned earlier, variance is highly sensitive to outliers. Extreme values can disproportionately inflate the variance, potentially misrepresenting the overall spread of the data. Robust measures of dispersion, such as the interquartile range, are less sensitive to outliers.
-
Units: The squared units of variance can be difficult to interpret directly, leading to the preference for the standard deviation in many practical applications.
-
Assumption of Normality: Many statistical tests that utilize variance assume that the data is normally distributed. If the data is significantly non-normal, the interpretation of variance might be less reliable. Transformations of the data or non-parametric methods might be necessary.
Conclusion: The Importance of Variance in Statistics
The square of the standard deviation, the variance, is a fundamental concept in statistics that quantifies the dispersion of a dataset around its mean. While the standard deviation provides a more intuitive measure of spread, the variance holds significant mathematical advantages and is essential for many advanced statistical techniques. Understanding variance is crucial for interpreting statistical results, building robust models, and making informed decisions in various fields, from finance and quality control to scientific research and machine learning. Its limitations, particularly sensitivity to outliers, should be considered when interpreting its value, especially in the presence of extreme data points. Always consider the context and the nature of your data when utilizing and interpreting variance in your analysis.
Latest Posts
Latest Posts
-
Find The Point On The Y Axis Which Is Equidistant From
May 09, 2025
-
Is 3 4 Bigger Than 7 8
May 09, 2025
-
Which Of These Is Not A Prime Number
May 09, 2025
-
What Is 30 Percent Off Of 80 Dollars
May 09, 2025
-
Are Alternate Exterior Angles Always Congruent
May 09, 2025
Related Post
Thank you for visiting our website which covers about The Square Of The Standard Deviation Is Called The . We hope the information provided has been useful to you. Feel free to contact us if you have any questions or need further assistance. See you next time and don't miss to bookmark.