What Does Area Under The Curve Mean In Statistics
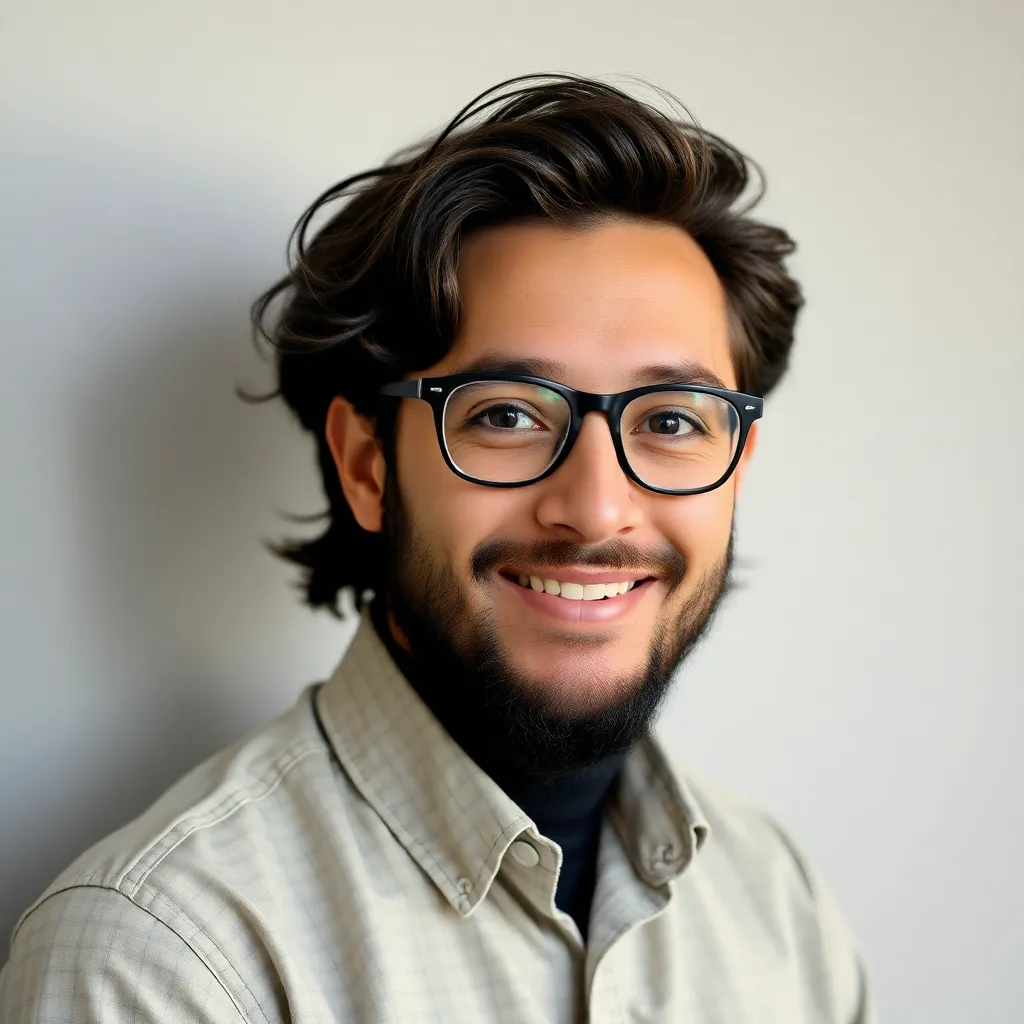
News Co
May 09, 2025 · 6 min read
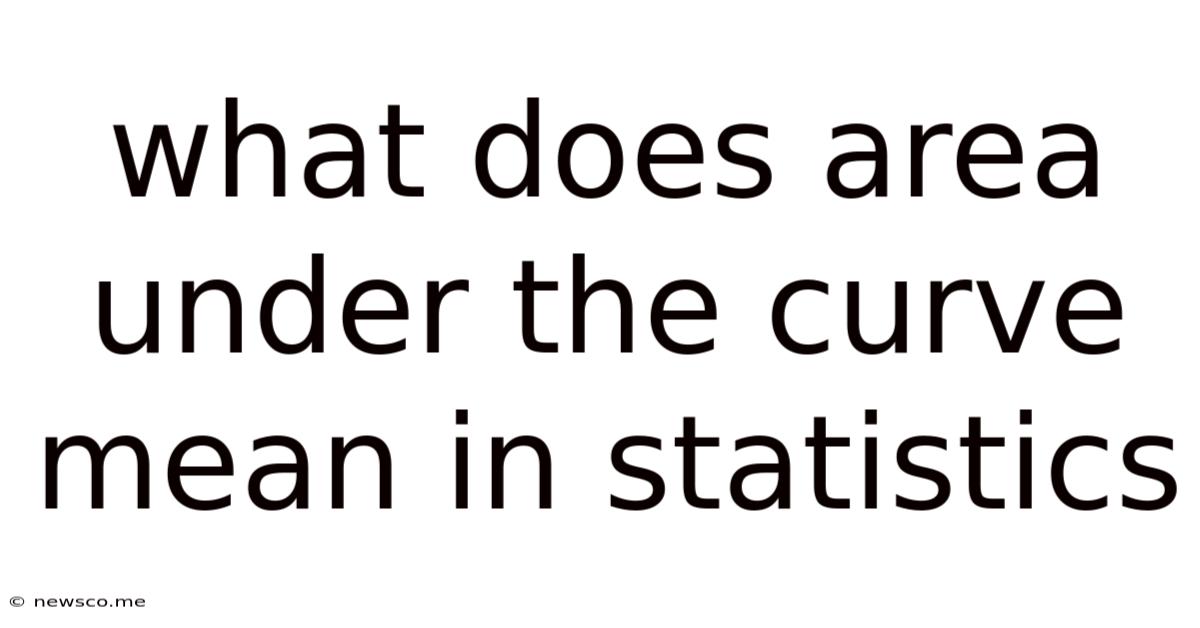
Table of Contents
- What Does Area Under The Curve Mean In Statistics
- Table of Contents
- What Does Area Under the Curve Mean in Statistics? A Comprehensive Guide
- Understanding the Concept of Area Under the Curve
- AUC Values and Their Interpretation
- Calculating the Area Under the Curve
- 1. Trapezoidal Rule
- 2. Mann-Whitney U Statistic
- 3. Numerical Integration Methods
- Applications of Area Under the Curve
- 1. Machine Learning and Classification
- 2. Medical Diagnosis and Prognosis
- 3. Credit Risk Assessment
- 4. Environmental Science
- 5. Information Retrieval
- Advantages and Limitations of AUC
- Conclusion
- Latest Posts
- Related Post
What Does Area Under the Curve Mean in Statistics? A Comprehensive Guide
The area under the curve (AUC) is a fundamental concept in statistics, particularly in the context of probability distributions and evaluating the performance of classification models. While seemingly simple, its implications are far-reaching, impacting various fields from medicine and finance to machine learning and environmental science. This comprehensive guide will delve into the meaning of AUC, its calculation, interpretation, and applications across different domains.
Understanding the Concept of Area Under the Curve
At its core, the AUC represents the probability that a randomly chosen positive observation will be ranked higher than a randomly chosen negative observation. Imagine you're building a model to predict whether a customer will click on an advertisement (positive) or not (negative). The AUC measures how well your model separates these two groups. A higher AUC indicates better separation, implying a more accurate predictive model.
Let's visualize this:
- The Curve: The "curve" refers to the Receiver Operating Characteristic (ROC) curve, a graphical representation of the trade-off between a model's true positive rate (TPR) and false positive rate (FPR) at various threshold settings.
- True Positive Rate (TPR) or Sensitivity: The proportion of actual positives that are correctly identified as such. It's the ratio of true positives to the total number of actual positives.
- False Positive Rate (FPR) or 1 - Specificity: The proportion of actual negatives that are incorrectly identified as positives. It's the ratio of false positives to the total number of actual negatives.
The ROC curve plots TPR against FPR for different classification thresholds. The AUC is the area enclosed by this curve and the x-axis.
AUC Values and Their Interpretation
The AUC value ranges from 0 to 1:
- AUC = 1: Perfect classifier. The model correctly classifies all positive and negative instances. The ROC curve will follow the top-left corner of the graph.
- AUC = 0.5: No discrimination. The model's performance is equivalent to random guessing. The ROC curve will be a diagonal line.
- AUC > 0.5: The model performs better than random guessing. A higher AUC value indicates better performance.
- AUC < 0.5: The model performs worse than random guessing. This is usually an indication of a problem with the model or the data. Inverting the predictions (i.e., switching positive and negative labels) will yield an AUC > 0.5.
It's important to note that while AUC provides a summary measure of model performance, it doesn't capture all aspects. For instance, a model with a high AUC might still have an unacceptable number of false positives in a specific application. Therefore, it's crucial to consider other metrics like precision, recall, and F1-score along with AUC for a comprehensive evaluation.
Calculating the Area Under the Curve
There are several ways to calculate the AUC:
1. Trapezoidal Rule
This is a common and relatively simple method. The ROC curve is approximated as a series of trapezoids, and the area of each trapezoid is calculated and summed. This approach is particularly useful when dealing with discrete data points.
The formula for the area of a single trapezoid is:
Area = 0.5 * (x₂ - x₁) * (y₁ + y₂)
where:
- x₁, x₂ are the x-coordinates (FPR)
- y₁, y₂ are the y-coordinates (TPR)
The total AUC is the sum of the areas of all trapezoids.
2. Mann-Whitney U Statistic
This non-parametric statistical test can also be used to compute the AUC. It measures the probability that a randomly selected observation from the positive class will have a higher score than a randomly selected observation from the negative class. The AUC is directly related to the U statistic:
AUC = U / (n₁ * n₂)
where:
- U is the Mann-Whitney U statistic
- n₁ is the number of positive instances
- n₂ is the number of negative instances
3. Numerical Integration Methods
For smoother ROC curves, numerical integration techniques like Simpson's rule or Gaussian quadrature can provide more accurate AUC estimations. These methods involve approximating the integral of the ROC curve using more sophisticated mathematical techniques.
Applications of Area Under the Curve
The AUC finds widespread application across various disciplines:
1. Machine Learning and Classification
AUC is a critical metric for evaluating the performance of binary classifiers. It's particularly useful when the classes are imbalanced (e.g., fraud detection, medical diagnosis). In such scenarios, accuracy alone can be misleading, while AUC provides a more robust measure of the model's ability to distinguish between the classes.
2. Medical Diagnosis and Prognosis
AUC is frequently employed to assess the diagnostic accuracy of medical tests. For example, the AUC of a blood test for a specific disease reflects how well the test differentiates between individuals with and without the disease. A higher AUC suggests a more accurate and reliable diagnostic tool. Similarly, it can be used in prognostic models, assessing the ability of a model to predict future outcomes such as patient survival.
3. Credit Risk Assessment
In finance, AUC is used to evaluate the performance of credit scoring models. It measures how well the model separates good borrowers (low risk) from bad borrowers (high risk). A higher AUC indicates a more effective model in predicting loan defaults.
4. Environmental Science
AUC is used in ecological studies to evaluate the performance of models predicting species occurrence or habitat suitability. It helps determine how well a model separates areas where a species is present from areas where it's absent.
5. Information Retrieval
In information retrieval systems, AUC can be used to evaluate the effectiveness of a search algorithm. It measures the ability of the algorithm to rank relevant documents higher than irrelevant documents.
Advantages and Limitations of AUC
Advantages:
- Robust to class imbalance: Unlike accuracy, AUC is not heavily influenced by the proportion of positive and negative instances in the dataset.
- Comprehensive performance measure: It summarizes the performance across all possible classification thresholds.
- Intuitive interpretation: The AUC value directly represents the probability of correctly ranking a positive instance higher than a negative instance.
- Wide applicability: It's used across various fields and applications.
Limitations:
- Doesn't consider costs of misclassification: It doesn't differentiate between the costs associated with false positives and false negatives, which can be crucial in some applications.
- Not suitable for multi-class problems: While extensions exist, AUC is primarily designed for binary classification.
- Can be misleading with highly skewed datasets: Although robust to class imbalance, extreme skewness might still affect interpretation.
- Doesn't provide insights into specific threshold performance: While providing an overall summary, it doesn't tell you the performance at a specific threshold.
Conclusion
The area under the curve is a powerful and versatile metric for evaluating the performance of classification models and assessing the discrimination ability of various tests and models across diverse fields. While it provides a valuable summary of model performance, it's crucial to interpret it in conjunction with other relevant metrics and consider its limitations within the specific context of the application. Understanding the calculation, interpretation, and applications of AUC is vital for anyone working with statistical modeling and data analysis. By carefully considering the strengths and weaknesses of AUC, researchers and practitioners can make informed decisions about the suitability of this metric in their specific applications and draw more accurate and meaningful conclusions from their data.
Latest Posts
Related Post
Thank you for visiting our website which covers about What Does Area Under The Curve Mean In Statistics . We hope the information provided has been useful to you. Feel free to contact us if you have any questions or need further assistance. See you next time and don't miss to bookmark.