What Is The Third Quartile Of This Data Set
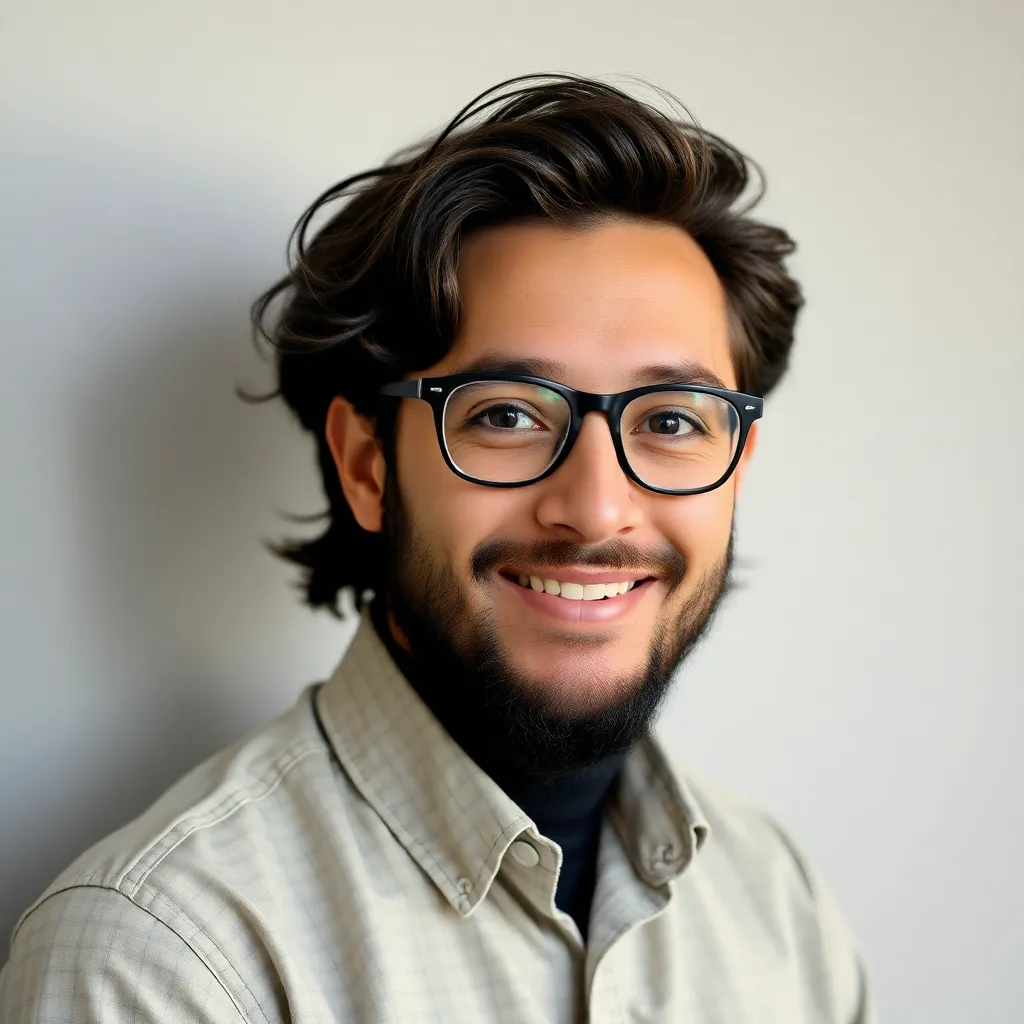
News Co
May 09, 2025 · 6 min read
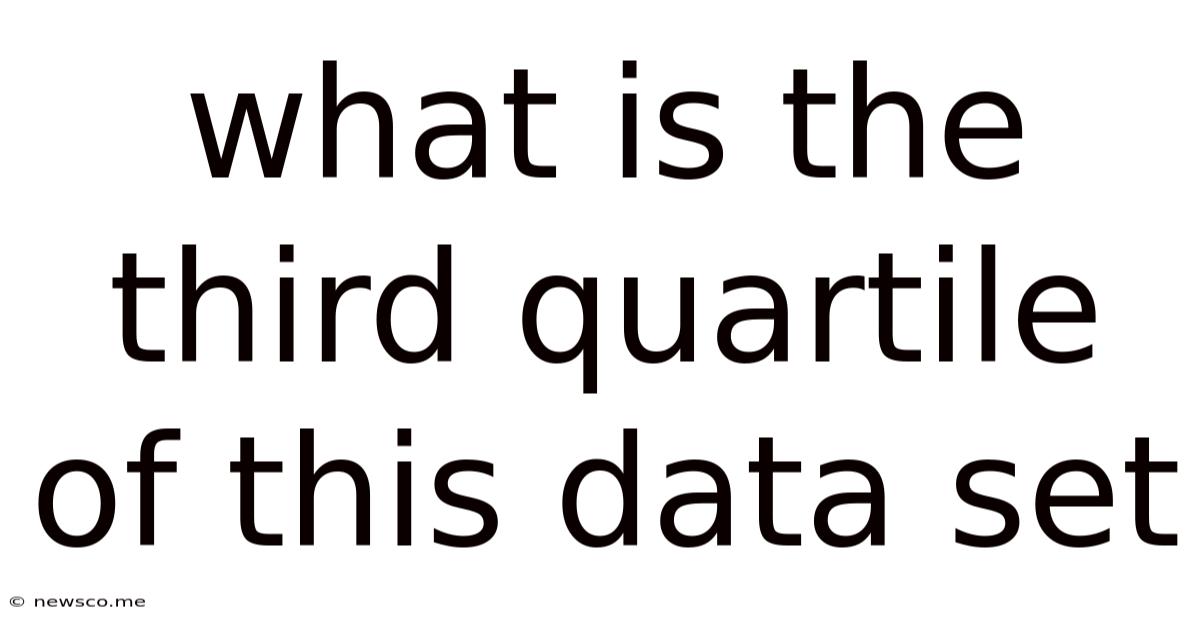
Table of Contents
What is the Third Quartile of This Data Set? A Comprehensive Guide
Understanding quartiles is crucial in descriptive statistics. They help us break down data into meaningful chunks, revealing the distribution and spread of our values. This article dives deep into the concept of the third quartile, explaining its calculation, significance, and application, using various examples to solidify your understanding. We'll explore different methods of calculation and tackle potential complexities.
What are Quartiles?
Before we delve into the third quartile specifically, let's establish a foundation by understanding what quartiles are. Quartiles are values that divide a dataset into four equal parts. Imagine you have a dataset sorted from smallest to largest; the quartiles are the points that split the data into 25% segments.
- First Quartile (Q1): Represents the 25th percentile. 25% of the data falls below Q1.
- Second Quartile (Q2): This is also known as the median. It represents the 50th percentile, with 50% of the data falling below Q2.
- Third Quartile (Q3): Represents the 75th percentile. 75% of the data falls below Q3.
- Fourth Quartile (Q4): This is simply the maximum value in the dataset.
Calculating the Third Quartile (Q3): Different Approaches
There are several ways to calculate the third quartile, and the method you choose might depend slightly on the characteristics of your data and the tools you're using. Let's examine the most common approaches.
Method 1: Using the Formula for Odd and Even Number of Data Points
This is a widely used method that takes into account whether your dataset has an odd or even number of data points.
Step 1: Sort the Data
The first, and arguably most important, step is to arrange your data in ascending order (from smallest to largest). This is essential for accurate quartile calculation.
Step 2: Identify the Median (Q2)
Find the median (Q2) of the entire dataset. If the number of data points (n) is odd, the median is the middle value. If n is even, the median is the average of the two middle values.
Step 3: Calculate Q3 for Odd n
If you have an odd number of data points, the third quartile (Q3) is the median of the upper half of the data (excluding the median itself).
Step 4: Calculate Q3 for Even n
If you have an even number of data points, the third quartile (Q3) is the median of the upper half of the data. This upper half includes the larger of the two middle values (which were used to calculate the median).
Example 1: Odd Number of Data Points
Let's consider the following dataset: 2, 4, 6, 8, 10, 12, 14
- Sorted Data: The data is already sorted.
- Median (Q2): The median is 8.
- Upper Half: The upper half of the data is 10, 12, 14.
- Q3: The median of the upper half is 12. Therefore, Q3 = 12.
Example 2: Even Number of Data Points
Let's consider this dataset: 2, 4, 6, 8, 10, 12
- Sorted Data: The data is already sorted.
- Median (Q2): The median is (6 + 8) / 2 = 7.
- Upper Half: The upper half of the data is 8, 10, 12.
- Q3: The median of the upper half is 10. Therefore, Q3 = 10.
Method 2: Using Percentiles
Another approach involves directly calculating the position of the third quartile using percentiles. This method is particularly useful when dealing with larger datasets or when working with statistical software.
The formula is:
Position of Q3 = 0.75 * (n + 1)
Where 'n' is the number of data points. The result will usually be a decimal.
Step 1: Calculate the Position
Use the formula above to calculate the position of Q3.
Step 2: Interpret the Position
- If the position is a whole number: Q3 is the value at that position in the sorted dataset.
- If the position is a decimal: Q3 is the average of the values at the whole number below and above the calculated position.
Example 3: Using the Percentile Method
Let's revisit the dataset: 2, 4, 6, 8, 10, 12
- n = 6
- Position of Q3 = 0.75 * (6 + 1) = 5.25
- Interpretation: Since 5.25 is a decimal, Q3 is the average of the 5th and 6th values in the sorted dataset. Therefore, Q3 = (10 + 12) / 2 = 11.
Method 3: Using Statistical Software
Statistical software packages (like R, SPSS, Excel, Python with libraries like NumPy and Pandas) readily calculate quartiles. These tools often use sophisticated algorithms that handle various data types and sizes efficiently. These built-in functions typically use more robust methods to handle edge cases and outliers.
Significance and Interpretation of the Third Quartile
The third quartile is a valuable descriptive statistic offering several insights:
-
Data Distribution: Q3, along with Q1 and the median, provides a comprehensive picture of the data's distribution. The distance between Q3 and Q1 (the interquartile range) is particularly informative about the spread of the central 50% of the data. A large IQR suggests high variability, while a small IQR indicates a tighter clustering of data points.
-
Outlier Detection: Q3 is used in conjunction with Q1 to identify potential outliers. Values significantly above Q3 (often defined by a multiple of the IQR) are flagged as potential outliers, warranting further investigation.
-
Comparative Analysis: Comparing Q3 across different datasets allows for insightful comparisons of central tendencies and data variability.
-
Data Summarization: Q3 simplifies the representation of complex datasets by providing a concise summary of the upper portion of the distribution.
Applications of the Third Quartile
The third quartile finds applications in diverse fields:
-
Finance: Analyzing financial data, such as stock prices or investment returns. Q3 helps identify the higher-performing investments or assets.
-
Healthcare: Analyzing patient data, such as vital signs or lab results. Q3 can help identify patients with higher values of a particular measure.
-
Education: Analyzing student test scores, Q3 reveals the performance of the top 75% of students.
-
Engineering: Analyzing measurements of manufactured components. Q3 may show the upper bound of acceptable tolerances.
Handling Missing Data and Outliers
Missing data and outliers pose challenges in quartile calculations. Several strategies can be employed to address these issues:
-
Missing Data: Techniques like imputation (replacing missing values with estimated values) can be used. The choice of imputation method depends on the nature of the missing data.
-
Outliers: Outliers can significantly influence the value of Q3. One approach is to remove or transform outliers before calculating the quartiles. However, caution should be exercised, as removing outliers might distort the true characteristics of the dataset. Robust methods for quartile calculations are less sensitive to outliers than traditional methods.
Conclusion
The third quartile is a fundamental concept in descriptive statistics. Understanding its calculation, interpretation, and significance allows for better analysis and presentation of data. While various methods exist for calculating Q3, the underlying principle remains the same: dividing the sorted dataset into four equal parts, with Q3 representing the value below which 75% of the data lies. Mastering quartile calculation is an essential skill for anyone working with data analysis. Remember to always consider the context of your data and the appropriate method for your specific needs. The use of statistical software can simplify the process, especially when dealing with large or complex datasets.
Latest Posts
Latest Posts
-
Find The Point On The Y Axis Which Is Equidistant From
May 09, 2025
-
Is 3 4 Bigger Than 7 8
May 09, 2025
-
Which Of These Is Not A Prime Number
May 09, 2025
-
What Is 30 Percent Off Of 80 Dollars
May 09, 2025
-
Are Alternate Exterior Angles Always Congruent
May 09, 2025
Related Post
Thank you for visiting our website which covers about What Is The Third Quartile Of This Data Set . We hope the information provided has been useful to you. Feel free to contact us if you have any questions or need further assistance. See you next time and don't miss to bookmark.