Which Correlation Coefficient Indicates The Strongest Relationship
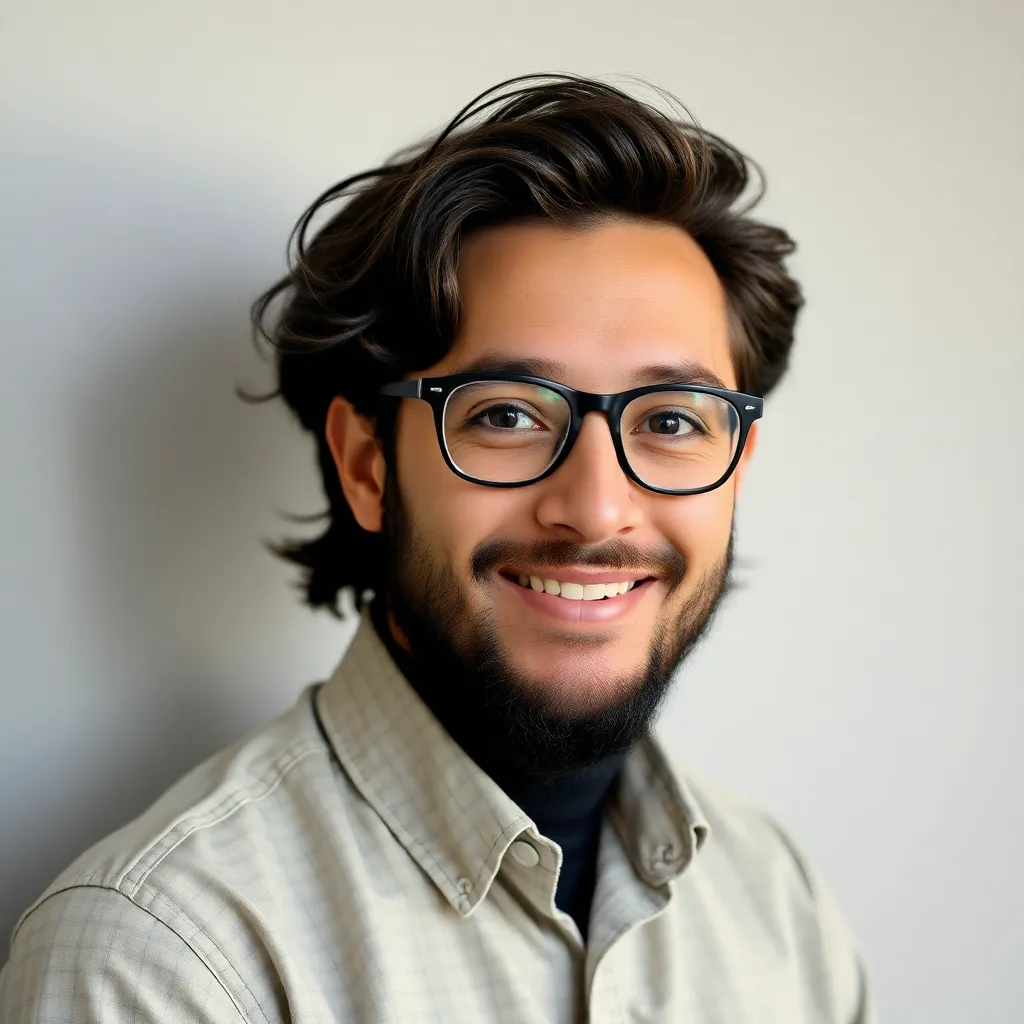
News Co
Mar 14, 2025 · 6 min read

Table of Contents
Which Correlation Coefficient Indicates the Strongest Relationship?
Understanding correlation coefficients is crucial for anyone working with data analysis, statistics, or research. These coefficients provide a quantifiable measure of the strength and direction of a linear relationship between two variables. But which correlation coefficient signifies the strongest relationship? This article dives deep into the world of correlation, exploring different types of coefficients and explaining how to interpret their values to identify the strongest associations.
Understanding Correlation Coefficients
Correlation coefficients are statistical measures that quantify the association between two variables. They range from -1 to +1, with the magnitude indicating the strength of the relationship and the sign indicating the direction.
- Positive Correlation (+1): A perfect positive correlation means that as one variable increases, the other increases proportionally. They move in the same direction.
- Negative Correlation (-1): A perfect negative correlation signifies that as one variable increases, the other decreases proportionally. They move in opposite directions.
- Zero Correlation (0): A zero correlation indicates no linear relationship between the variables. Changes in one variable do not predict changes in the other.
However, it's important to remember that correlation does not imply causation. Even a strong correlation doesn't prove that one variable causes changes in the other. There could be other underlying factors or confounding variables at play.
Types of Correlation Coefficients
Several correlation coefficients exist, each suited for different types of data:
1. Pearson's r (Product-Moment Correlation)
This is the most commonly used correlation coefficient and measures the linear association between two continuous variables. It assumes that the data is normally distributed and follows a linear relationship. A Pearson's r of +1 or -1 indicates a perfect linear correlation, while a value of 0 indicates no linear correlation. The closer the absolute value of Pearson's r is to 1, the stronger the linear relationship.
Example: Analyzing the relationship between height and weight. We'd expect a positive Pearson's r because taller individuals tend to weigh more.
2. Spearman's Rank Correlation (ρ)
Spearman's ρ is a non-parametric correlation coefficient, meaning it doesn't assume normality of the data. It measures the monotonic relationship between two variables. A monotonic relationship means that as one variable increases, the other variable tends to either increase or decrease consistently, but not necessarily linearly. This makes it suitable for ordinal data (ranked data) or continuous data that doesn't meet the assumptions of Pearson's r. Like Pearson's r, the closer the absolute value of Spearman's ρ is to 1, the stronger the monotonic relationship.
Example: Analyzing the relationship between exam rank and hours of study. Even if the relationship isn't perfectly linear, a strong positive Spearman's ρ would indicate that higher study hours generally lead to better exam ranks.
3. Kendall's Tau (τ)
Another non-parametric correlation coefficient, Kendall's Tau, measures the ordinal association between two variables. It's less sensitive to outliers than Spearman's ρ and is often preferred when dealing with smaller datasets or data with many tied ranks. The closer the absolute value of Kendall's Tau is to 1, the stronger the monotonic relationship.
Example: Analyzing the relationship between customer satisfaction ratings (ordinal data) and customer loyalty (ordinal data).
4. Point-Biserial Correlation
This correlation coefficient is used when one variable is continuous and the other is dichotomous (binary, having only two categories). It essentially measures the relationship between a continuous variable and a binary variable. The closer the absolute value is to 1, the stronger the relationship.
Example: Analyzing the relationship between test scores (continuous) and whether a student passed or failed the exam (dichotomous).
Interpreting Correlation Coefficients: Strength of Relationship
While the range of all these correlation coefficients is -1 to +1, interpreting the strength of the relationship isn't always about specific numerical thresholds. Context matters significantly. What might be considered a strong correlation in one field might be weak in another. However, some general guidelines are commonly used:
- 0.00-0.19: Very weak or no correlation
- 0.20-0.39: Weak correlation
- 0.40-0.59: Moderate correlation
- 0.60-0.79: Strong correlation
- 0.80-1.00: Very strong correlation
These are just guidelines, and the practical significance of the correlation strength depends entirely on the research context. A correlation of 0.4 might be considered substantial in one study but weak in another.
Choosing the Right Correlation Coefficient
Selecting the appropriate correlation coefficient depends on the type of data you're working with and the assumptions you're willing to make.
- Continuous, normally distributed data with a linear relationship: Use Pearson's r.
- Ordinal data or continuous data that doesn't meet assumptions of Pearson's r: Use Spearman's ρ or Kendall's Tau.
- One continuous and one dichotomous variable: Use point-biserial correlation.
Beyond Correlation: Exploring Causation and Other Relationships
It's crucial to emphasize again that correlation does not equal causation. A strong correlation doesn't prove a causal relationship. To establish causality, more rigorous methods like controlled experiments or longitudinal studies are necessary.
Furthermore, correlation coefficients only capture linear relationships. Two variables might have a strong non-linear relationship that a correlation coefficient might not detect. Visualizing your data with scatter plots is crucial to identify potential non-linear patterns.
Advanced Considerations and Limitations
- Outliers: Outliers can significantly influence correlation coefficients, particularly Pearson's r. Careful examination and potential removal (with justification) of outliers might be necessary.
- Sample Size: A larger sample size generally leads to more reliable correlation estimates. Small sample sizes can lead to inflated or deflated correlation coefficients.
- Restricted Range: If the range of values for one or both variables is restricted, the correlation coefficient might underestimate the true strength of the relationship.
- Heteroscedasticity: This refers to the unequal variance of the data points along the regression line. It can affect the reliability of correlation coefficients, especially Pearson's r.
Conclusion: Identifying the Strongest Relationship
The strongest relationship is indicated by a correlation coefficient closest to either +1 or -1, depending on whether the relationship is positive or negative. However, the "strength" is relative to the context and the type of data being analyzed. Choosing the right correlation coefficient based on the data type and understanding its limitations is crucial for accurate interpretation. Always visualize your data and consider potential confounding factors before drawing conclusions about the strength and nature of the relationship between variables. Remember that a strong correlation doesn't necessarily imply causation, and exploring other analytical methods may be necessary to fully understand the relationship between your variables. Always contextualize your findings and be mindful of potential biases or limitations within your data and methodology.
Latest Posts
Latest Posts
-
Find The Point On The Y Axis Which Is Equidistant From
May 09, 2025
-
Is 3 4 Bigger Than 7 8
May 09, 2025
-
Which Of These Is Not A Prime Number
May 09, 2025
-
What Is 30 Percent Off Of 80 Dollars
May 09, 2025
-
Are Alternate Exterior Angles Always Congruent
May 09, 2025
Related Post
Thank you for visiting our website which covers about Which Correlation Coefficient Indicates The Strongest Relationship . We hope the information provided has been useful to you. Feel free to contact us if you have any questions or need further assistance. See you next time and don't miss to bookmark.