Which Of The Following Are Example Of Inferential Statistics
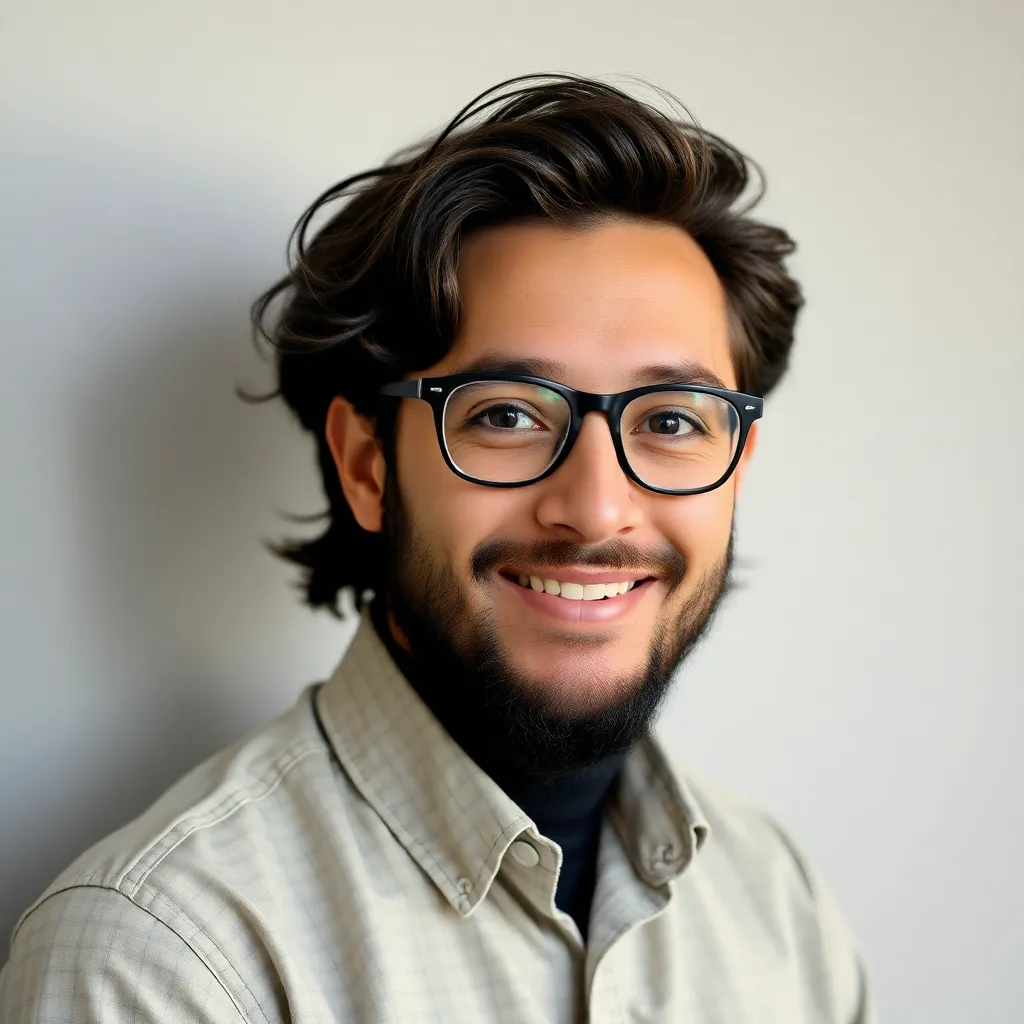
News Co
May 04, 2025 · 7 min read

Table of Contents
Which of the Following are Examples of Inferential Statistics?
Inferential statistics is a powerful branch of statistics that allows us to draw conclusions about a population based on a sample of data. Unlike descriptive statistics, which simply summarize data, inferential statistics uses sample data to make inferences about broader trends and characteristics. This involves estimating population parameters, testing hypotheses, and making predictions. Understanding the distinction is crucial for anyone working with data analysis. This comprehensive guide will delve into what constitutes inferential statistics, explore various examples, and highlight the critical differences between inferential and descriptive statistics.
Understanding Inferential Statistics
Inferential statistics employs probability theory to make generalizations about a population from a smaller subset, the sample. The core principle lies in the assumption that the sample accurately reflects the population. This assumption is crucial because analyzing an entire population is often impractical, expensive, or even impossible. Therefore, we rely on meticulously selected samples to draw meaningful conclusions.
The process generally involves these key steps:
-
Collecting Data: Gathering data from a representative sample of the population. The sampling method is crucial here to avoid bias and ensure the sample accurately represents the population.
-
Analyzing Data: Using statistical tests and techniques to analyze the sample data. This involves calculating descriptive statistics (like mean, median, and standard deviation) but goes beyond that to perform inferential tests.
-
Making Inferences: Drawing conclusions about the population based on the sample analysis. This is where we move beyond simply describing the data to making claims about the broader group. These conclusions are expressed with a degree of uncertainty, typically represented by a confidence interval or p-value.
-
Drawing Conclusions: Based on the inferential analysis, researchers can accept or reject hypotheses, make predictions, or estimate population parameters.
Key Techniques in Inferential Statistics
Several techniques fall under the umbrella of inferential statistics. These techniques provide different ways to analyze data and draw inferences, each suitable for different research questions and data types. Some of the most common include:
1. Hypothesis Testing:
Hypothesis testing is a cornerstone of inferential statistics. It involves formulating a null hypothesis (a statement of no effect or difference) and an alternative hypothesis (a statement contradicting the null hypothesis). Statistical tests are then used to determine whether there is enough evidence to reject the null hypothesis in favor of the alternative. Common hypothesis tests include:
-
t-tests: Used to compare the means of two groups. Independent samples t-tests compare the means of two independent groups, while paired samples t-tests compare the means of two related groups (e.g., before-and-after measurements).
-
ANOVA (Analysis of Variance): Used to compare the means of three or more groups. ANOVA can detect differences between group means while controlling for multiple comparisons.
-
Chi-Square Test: Used to analyze categorical data and determine if there is a significant association between two categorical variables.
-
Z-tests: Used to test hypotheses about population means when the population standard deviation is known or the sample size is large.
2. Confidence Intervals:
Confidence intervals provide a range of values within which a population parameter (e.g., the mean) is likely to fall. They express the uncertainty associated with estimating the population parameter from a sample. A 95% confidence interval, for instance, means that if we were to repeat the sampling process many times, 95% of the calculated confidence intervals would contain the true population parameter.
3. Regression Analysis:
Regression analysis examines the relationship between a dependent variable and one or more independent variables. It allows us to predict the value of the dependent variable based on the values of the independent variables. Different types of regression analysis exist, including:
-
Linear Regression: Used when the relationship between variables is linear.
-
Multiple Regression: Used when there are multiple independent variables.
-
Logistic Regression: Used when the dependent variable is categorical.
4. Correlation Analysis:
Correlation analysis measures the strength and direction of the linear relationship between two variables. The correlation coefficient (often denoted as 'r') ranges from -1 to +1, with -1 indicating a perfect negative correlation, +1 indicating a perfect positive correlation, and 0 indicating no linear correlation.
Examples of Inferential Statistics in Action
Let's explore concrete examples to solidify our understanding.
Example 1: A Pharmaceutical Trial
A pharmaceutical company conducts a clinical trial to test the effectiveness of a new drug for lowering blood pressure. They randomly assign participants to either a treatment group (receiving the new drug) or a control group (receiving a placebo). After a certain period, they measure the blood pressure of participants in both groups. Using a t-test, they compare the average blood pressure reduction in the treatment group to that in the control group. If the difference is statistically significant (p-value < 0.05, for example), they can infer that the new drug is effective in lowering blood pressure. This is a clear application of hypothesis testing. They might also calculate a confidence interval for the difference in mean blood pressure reduction to estimate the range of effectiveness.
Example 2: Election Polling
Political pollsters survey a sample of registered voters to predict the outcome of an election. They ask voters about their voting intentions and analyze the results to estimate the proportion of voters who will support each candidate. This uses estimation techniques to infer the overall population voting preferences based on the sample data. They present their findings as percentages with a margin of error, which represents the uncertainty associated with their estimate (related to the confidence interval concept).
Example 3: Analyzing Customer Satisfaction
A company surveys a sample of its customers to assess their satisfaction with a new product. They use a questionnaire with rating scales and analyze the responses to determine the average customer satisfaction score. They may also analyze the relationship between customer satisfaction and other variables, such as age, gender, or purchase history using correlation or regression analysis. This allows them to make inferences about the overall customer satisfaction with the product and identify factors that influence satisfaction. This example demonstrates inferential statistics applied to market research.
Example 4: Studying the Impact of Education on Income
Researchers might collect data on individuals' years of education and their annual income. They can then use regression analysis to investigate the relationship between education and income. The results will allow them to infer whether there's a statistically significant relationship and, if so, the strength and direction of that relationship. This is a broader social science application of inferential statistics.
Example 5: Assessing the Effectiveness of a New Teaching Method
A school district wants to evaluate the effectiveness of a new teaching method. They randomly assign students to either the traditional method or the new method. After a set time, both groups take the same test, and the results are analyzed using an independent samples t-test or ANOVA to determine whether the new method significantly improves student performance. This is another application of hypothesis testing.
Inferential vs. Descriptive Statistics: Key Differences
It's crucial to distinguish between inferential and descriptive statistics. While both are vital in data analysis, they serve different purposes:
Feature | Descriptive Statistics | Inferential Statistics |
---|---|---|
Purpose | Summarizes and describes data | Makes inferences and predictions about a population |
Data | Uses the entire dataset or a sample | Primarily uses sample data |
Techniques | Mean, median, mode, standard deviation, frequency tables | Hypothesis testing, confidence intervals, regression analysis |
Output | Numerical summaries and visualizations of data | Probabilistic statements about population parameters |
Goal | Organize and present data | Generalize from a sample to a population |
Conclusion
Inferential statistics provides powerful tools for drawing meaningful conclusions from data. By understanding the various techniques and their applications, researchers and analysts can gain valuable insights into population characteristics, test hypotheses, and make informed decisions. The examples provided illustrate the breadth of applications across various fields, highlighting the versatility and importance of inferential statistics in modern data analysis. From pharmaceutical trials to election polling and market research, its influence is pervasive and crucial for evidence-based decision making. Remember that careful sample selection and appropriate statistical techniques are essential for reliable inferences.
Latest Posts
Latest Posts
-
What Triangle Has Two Equal Sides
May 05, 2025
-
How Many Millimeters In 5 Liters
May 05, 2025
-
Half Of 1 3 4 Cup Of Flour
May 05, 2025
-
Whats 25 Percent Off Of 60
May 05, 2025
-
What Is A Quadrilateral With No Right Angles
May 05, 2025
Related Post
Thank you for visiting our website which covers about Which Of The Following Are Example Of Inferential Statistics . We hope the information provided has been useful to you. Feel free to contact us if you have any questions or need further assistance. See you next time and don't miss to bookmark.