Which R-value Represents The Strongest Correlation 0.83 0.67 0.48 0.79
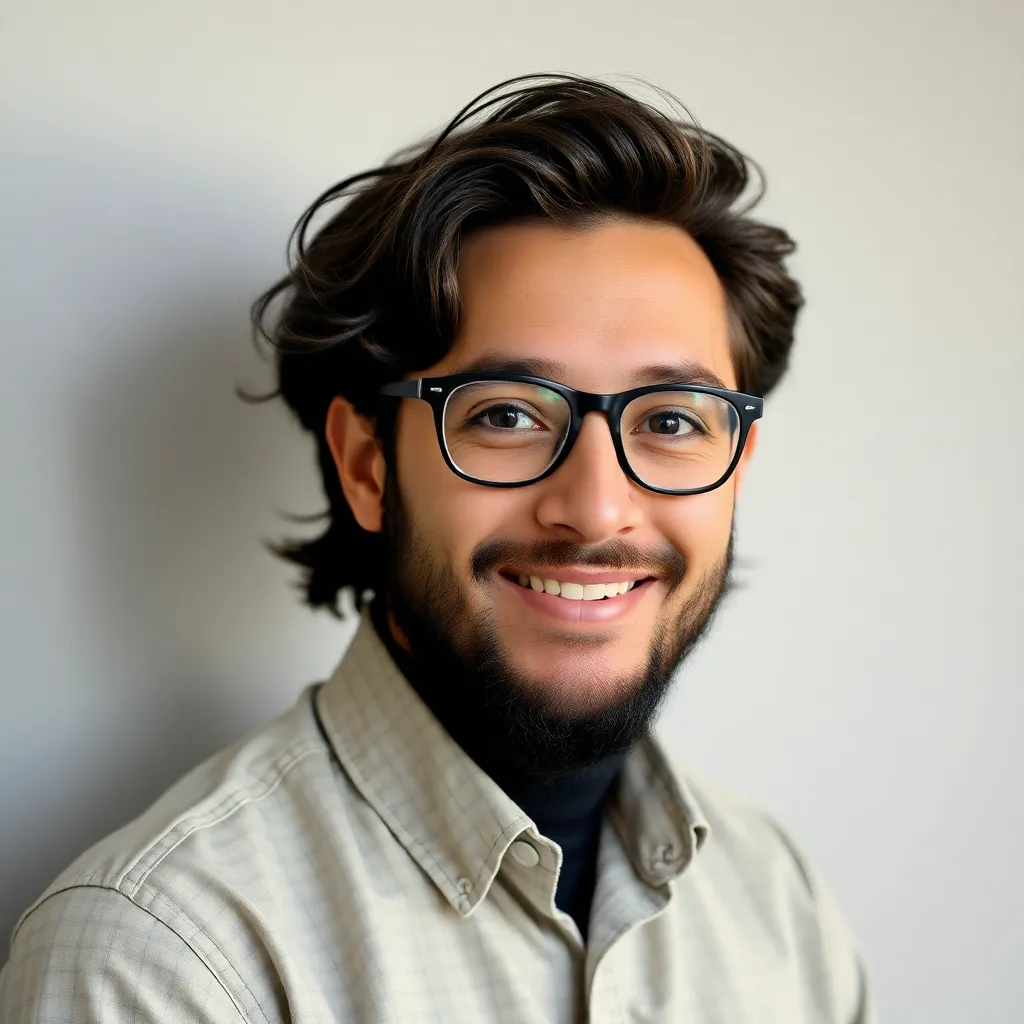
News Co
May 08, 2025 · 5 min read
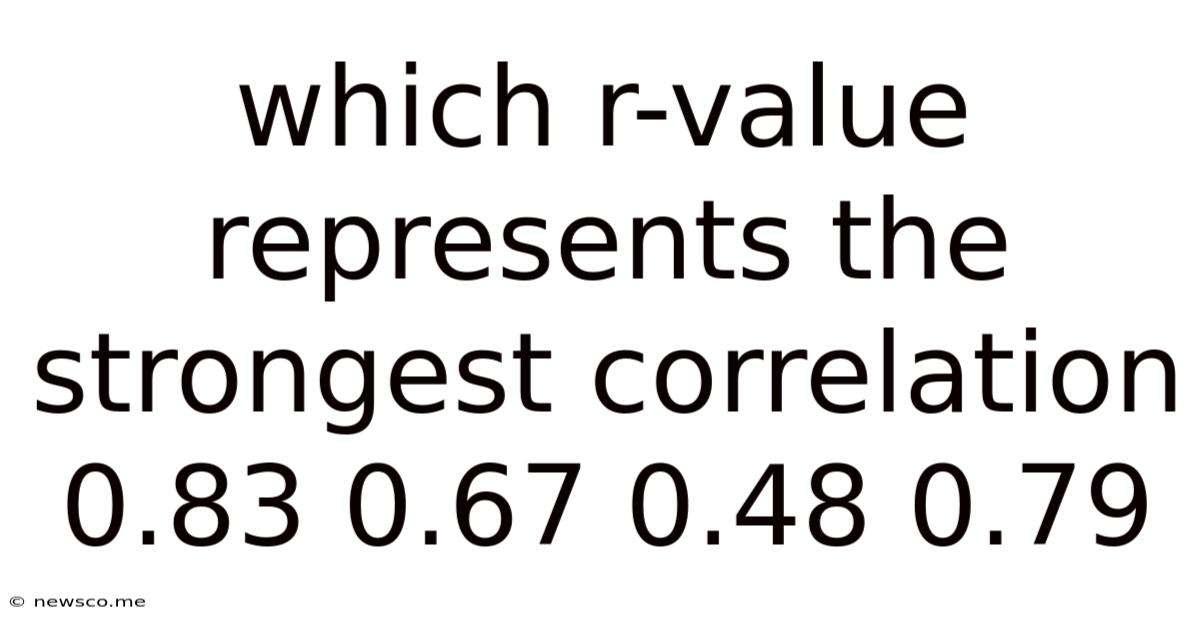
Table of Contents
Which R-Value Represents the Strongest Correlation: 0.83, 0.67, 0.48, or 0.79? Understanding Correlation Coefficients
Correlation is a fundamental concept in statistics that measures the strength and direction of a linear relationship between two variables. Understanding correlation is crucial in various fields, from social sciences and finance to engineering and medicine. This article delves into the meaning of correlation coefficients, specifically the r-value, and determines which of the given values (0.83, 0.67, 0.48, and 0.79) represents the strongest correlation. We'll also explore the interpretation of different r-values and their practical implications.
Understanding the Correlation Coefficient (r-value)
The correlation coefficient, often denoted as r, is a standardized measure ranging from -1 to +1. It quantifies the linear association between two variables:
- +1: Indicates a perfect positive correlation. As one variable increases, the other increases proportionally.
- 0: Indicates no linear correlation. There's no linear relationship between the variables. Note that this doesn't necessarily mean there's no relationship at all; it simply means there's no linear relationship. A non-linear relationship might still exist.
- -1: Indicates a perfect negative correlation. As one variable increases, the other decreases proportionally.
Values between -1 and +1 represent varying degrees of correlation strength. The closer the absolute value of r is to 1, the stronger the linear correlation. The sign (+ or -) simply indicates the direction of the relationship.
Interpreting the Given R-Values
Now let's analyze the provided r-values: 0.83, 0.67, 0.48, and 0.79. To determine which represents the strongest correlation, we simply look at the absolute values:
- |0.83| = 0.83
- |0.67| = 0.67
- |0.48| = 0.48
- |0.79| = 0.79
Clearly, 0.83 represents the strongest correlation among the given options. This indicates a strong positive linear relationship between the two variables.
Strength of Correlation: A Detailed Look
While we've identified 0.83 as the strongest, it's helpful to understand the general interpretation of correlation strength:
- |r| ≥ 0.8: Indicates a very strong correlation. Changes in one variable are strongly associated with changes in the other.
- 0.6 ≤ |r| < 0.8: Indicates a strong correlation. A noticeable relationship exists, but it's not as precise as a very strong correlation.
- 0.4 ≤ |r| < 0.6: Indicates a moderate correlation. A discernible relationship is present, but it's weaker than a strong correlation. Other factors likely influence the variables.
- 0.2 ≤ |r| < 0.4: Indicates a weak correlation. The relationship is subtle, and other factors likely play a more significant role.
- |r| < 0.2: Indicates a very weak or negligible correlation. There's little to no linear relationship between the variables.
Based on this scale, we can classify the given r-values:
- 0.83: Very strong positive correlation
- 0.79: Strong positive correlation
- 0.67: Strong positive correlation
- 0.48: Moderate positive correlation
Beyond the R-Value: Important Considerations
While the r-value is a useful measure, it's crucial to remember several limitations:
1. Correlation Does Not Imply Causation
This is perhaps the most important caveat. A high correlation coefficient only indicates an association between two variables; it doesn't prove that one variable causes changes in the other. There might be a third, unobserved variable influencing both. For example, a strong correlation between ice cream sales and drowning incidents doesn't mean ice cream consumption causes drowning. Both are likely influenced by a third variable: hot weather.
2. Linearity Assumption
The r-value measures linear correlation. If the relationship between the variables is non-linear (e.g., curved), the r-value might underestimate the true strength of the association. Scatter plots are essential to visually assess the relationship and determine if a linear model is appropriate.
3. Outliers' Influence
Outliers (extreme data points) can significantly influence the r-value. A single outlier can artificially inflate or deflate the correlation coefficient. Careful examination of the data for outliers is essential.
4. Sample Size
The reliability of the r-value increases with a larger sample size. A high r-value based on a small sample might not be as reliable as a similar r-value based on a large sample.
5. Spurious Correlation
Sometimes, a high correlation can be purely coincidental. This is known as spurious correlation. It's crucial to consider the context and potential underlying mechanisms before drawing conclusions based solely on the r-value.
Practical Applications and Examples
Correlation analysis is widely used across various fields:
- Finance: Assessing the relationship between stock prices and market indices.
- Economics: Studying the relationship between inflation and unemployment.
- Medicine: Investigating the association between lifestyle factors and disease risk.
- Social Sciences: Examining the correlation between education levels and income.
- Engineering: Analyzing the relationship between material properties and performance.
In each case, understanding the strength and direction of the correlation provides valuable insights, allowing for better predictions, informed decision-making, and the development of more accurate models. However, always remember the limitations discussed above – correlation doesn't equal causation, and other factors need to be carefully considered.
Conclusion: Interpreting Correlation Wisely
Determining which r-value represents the strongest correlation is straightforward: the higher the absolute value, the stronger the correlation. In this case, 0.83 represents the strongest correlation. However, interpreting correlation coefficients requires a nuanced understanding of their limitations. Always consider the context, visualize the data using scatter plots, be aware of potential outliers and non-linear relationships, and remember that correlation does not imply causation. By applying these principles, you can effectively use correlation analysis to gain meaningful insights from your data. The r-value provides a valuable quantitative measure, but it's only one piece of the puzzle in understanding the relationships between variables. Further investigation and a holistic approach are always necessary for robust conclusions.
Latest Posts
Latest Posts
-
True Or False All Rational Numbers Are Integers
May 09, 2025
-
Which Ratio Is Equivalent To The Ratio 2 5
May 09, 2025
-
10 Is 40 Of What Number
May 09, 2025
-
Which Rule Describes The Function In The Graph Below
May 09, 2025
-
Can A Scalene Triangle Be Obtuse
May 09, 2025
Related Post
Thank you for visiting our website which covers about Which R-value Represents The Strongest Correlation 0.83 0.67 0.48 0.79 . We hope the information provided has been useful to you. Feel free to contact us if you have any questions or need further assistance. See you next time and don't miss to bookmark.