Why Do You Split Stems On A Stem Plot
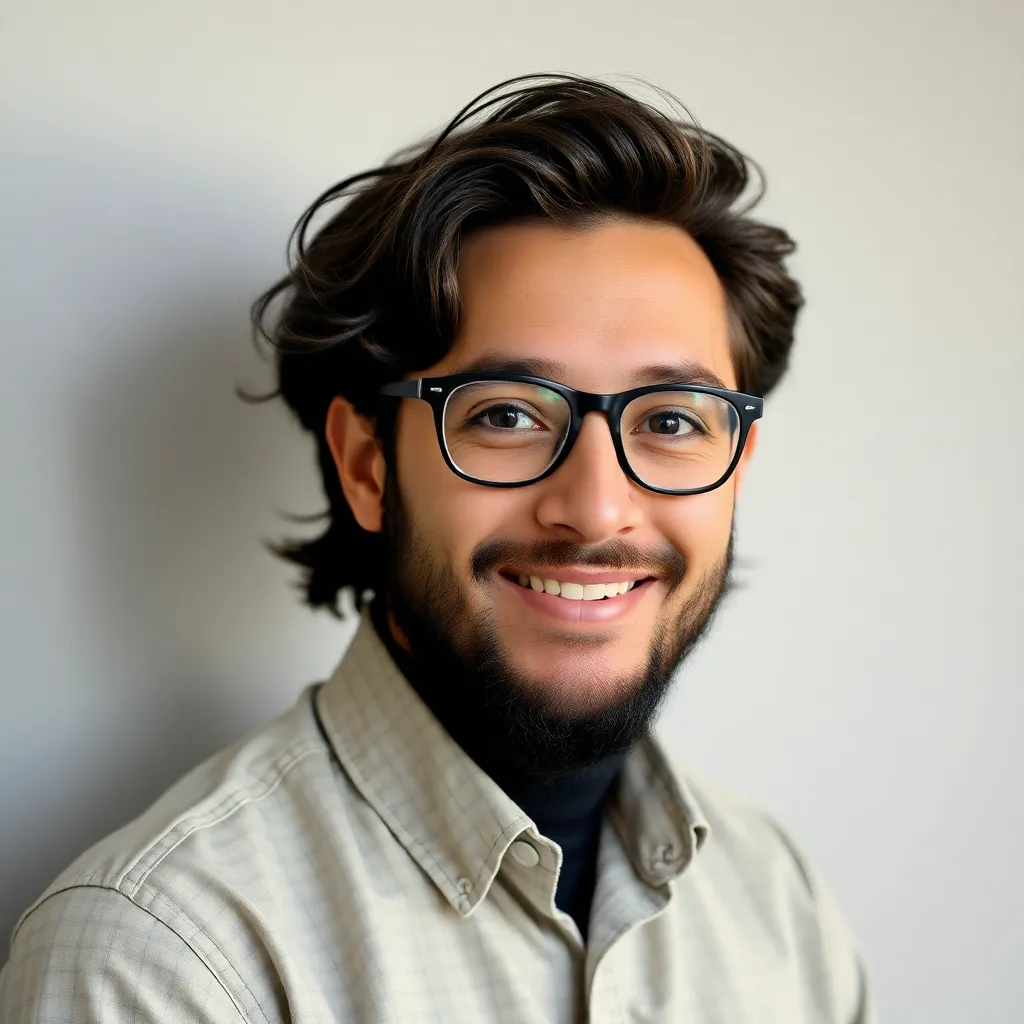
News Co
May 05, 2025 · 6 min read

Table of Contents
Why Do You Split Stems on a Stem Plot? A Deep Dive into Data Visualization
Stem plots, also known as stem-and-leaf plots, are a valuable tool for visualizing numerical data. They offer a unique blend of simplicity and informational richness, allowing for a quick grasp of data distribution, central tendency, and potential outliers. One crucial aspect of creating effective stem plots is understanding when and how to split stems. This practice significantly enhances the plot's readability and reveals finer details within the dataset. Let's delve into the reasons behind stem splitting and explore its impact on data analysis.
The Fundamentals of Stem Plots
Before diving into stem splitting, let's establish a foundational understanding of how stem plots work. A stem plot organizes data by separating each data point into two parts: a stem and a leaf. The stem represents the leading digit(s) of the data point, while the leaf represents the trailing digit(s). For instance, in a dataset containing the number 23, the stem would be 2 and the leaf would be 3.
Example: Consider the following dataset representing the ages of participants in a workshop: 22, 25, 28, 31, 31, 35, 39, 42, 45, 48, 51, 56.
A basic stem plot might look like this:
2 | 2 5 8
3 | 1 1 5 9
4 | 2 5 8
5 | 1 6
Here, the stems (2, 3, 4, 5) represent the tens digits, and the leaves represent the units digits. This simple plot provides a quick overview of the age distribution. However, notice how the data is somewhat clumped together, making it difficult to discern subtle variations in distribution. This is where stem splitting comes into play.
Why Split Stems? Improving Clarity and Detail
Stem splitting is a technique used to improve the resolution and clarity of a stem plot, especially when dealing with datasets that have a relatively narrow range or when the data points are densely clustered within a small range of values. The primary reasons for splitting stems are:
-
Increased Resolution: By splitting stems, we create more rows in the plot, thus spreading out the data points. This reduces the visual crowding and allows for a more nuanced understanding of the data distribution. Instead of having many leaves on a single stem, we distribute them across multiple stems, making individual data points more distinguishable.
-
Improved Visual Representation: A stem plot with split stems provides a clearer picture of the data's shape and spread. It can reveal patterns and clusters that might be missed in an unsplit stem plot. This improved visual clarity leads to more accurate interpretations and insights.
-
Enhanced Detection of Outliers and Clusters: Splitting stems makes it easier to spot outliers. When data points are tightly clustered, outliers stand out more prominently in a split stem plot because of the increased spread of data points. Similarly, subtle clusters of data that might be obscured in an unsplit plot become more apparent after splitting.
How to Split Stems: A Step-by-Step Guide
Splitting stems involves dividing each stem into two or more sub-stems. The most common approach is to split each stem in half. For example, if the stems represent tens digits, splitting them would mean creating sub-stems for 20-24 and 25-29. This process dramatically alters the plot's visual representation.
Let's revisit our age dataset and illustrate stem splitting:
Original Dataset: 22, 25, 28, 31, 31, 35, 39, 42, 45, 48, 51, 56
Unsplit Stem Plot:
2 | 2 5 8
3 | 1 1 5 9
4 | 2 5 8
5 | 1 6
Split Stem Plot (splitting each stem into two):
2 | 2
2 | 5 8
3 | 1 1
3 | 5 9
4 | 2 5
4 | 8
5 | 1
5 | 6
Notice how the split stem plot provides a much clearer picture of the data distribution. The data points are more spread out, making it easier to identify patterns and potential outliers. Each stem now represents a smaller range of values, resulting in a more refined visual representation.
Choosing the Right Splitting Strategy:
The decision on whether to split stems and how many times to do so depends entirely on the characteristics of the dataset.
-
Densely clustered data: If your data is heavily concentrated in a small range, splitting is highly recommended. This improves resolution and prevents overcrowding.
-
Sparsely distributed data: If your data is spread across a wide range with many gaps, splitting might be unnecessary and could even make the plot less informative.
-
Data range: The range of your data also plays a role. Wider ranges may not benefit as much from splitting as narrower ranges.
Advanced Stem Splitting Techniques
While splitting each stem in half is the most common method, other techniques can be employed depending on the data's characteristics:
-
Multiple Splits: For extremely dense datasets, you can split each stem into more than two sub-stems. For example, you could split each stem into thirds or quarters. This enhances resolution even further.
-
Unequal Splits: In certain cases, unequal splitting might be beneficial. For instance, you might split a stem at a significant data point or a natural break within the data.
Beyond Visual Representation: Analytical Benefits of Stem Splitting
Stem splitting isn't solely about aesthetics; it significantly aids in data analysis. The enhanced resolution and clearer visualization lead to better interpretations and a deeper understanding of the data's characteristics. Here's how:
-
More Accurate Measures of Central Tendency: With split stems, calculating the median and identifying the mode become easier and more precise. The improved data distribution clarity helps in pinpointing these central tendency measures accurately.
-
Improved Outlier Identification: As mentioned earlier, splitting stems makes outliers more noticeable. This is crucial for identifying potential data errors or exceptional cases that require further investigation.
-
Better Understanding of Data Skewness: The shape of the stem plot (after splitting) reveals more details about the data's skewness – whether it's symmetrically distributed, skewed to the left (negatively skewed), or skewed to the right (positively skewed).
-
Facilitates Comparisons: Stem plots with split stems are excellent for comparing two or more datasets visually. The enhanced resolution makes it easier to spot differences and similarities in their distribution and shape.
Conclusion: A Powerful Tool for Data Exploration
Stem splitting is a simple yet powerful technique that can substantially improve the effectiveness of stem plots. By increasing resolution, enhancing visual clarity, and promoting a more nuanced understanding of data distribution, stem splitting transforms a basic visualization tool into a more sophisticated analytical asset. The decision of whether or not to split stems should be guided by the specific dataset's properties, but when applied appropriately, this method offers significant advantages for exploratory data analysis. Remember, the goal is to create a stem plot that is both informative and easy to interpret, and stem splitting often proves to be the key to achieving this.
Latest Posts
Latest Posts
-
All The Sides Of A Triangle Equal
May 05, 2025
-
Find All Real Square Roots Of
May 05, 2025
-
Which Of The Following Is Greater
May 05, 2025
-
Difference Between Algebraic Expression And Algebraic Equation
May 05, 2025
-
Find The Area Of Shaded Portion Of Rectangle
May 05, 2025
Related Post
Thank you for visiting our website which covers about Why Do You Split Stems On A Stem Plot . We hope the information provided has been useful to you. Feel free to contact us if you have any questions or need further assistance. See you next time and don't miss to bookmark.